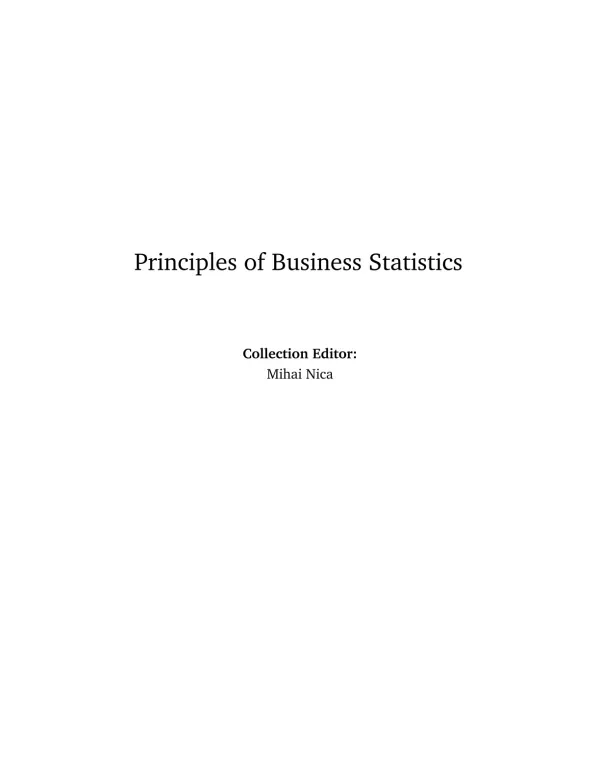
Business Statistics Principles
Document information
Author | Susan Dean |
instructor/editor | Mihai Nica |
subject/major | Business Statistics |
Document type | Textbook |
Language | English |
Format | |
Size | 1.49 MB |
Summary
I.Descriptive Statistics Summarizing and Visualizing Data
This section focuses on descriptive statistics, methods for summarizing and presenting data. Key concepts include measures of central tendency (mean, median, mode), measures of spread (variance, standard deviation, range), and various data visualization techniques like histograms, dot plots, bar charts, boxplots, and frequency polygons. Understanding these allows for effective data interpretation and initial insights into data characteristics. The use of histograms is particularly emphasized for larger datasets (100 values or more).
1. Introduction to Descriptive Statistics
The initial section establishes the core concept of descriptive statistics as the process of organizing and summarizing data. It contrasts this with inferential statistics, which uses probability to draw conclusions about populations. The text highlights the importance of understanding data, emphasizing that the goal is not just calculation but insightful interpretation. Practical applications are mentioned, such as analyzing data in newspapers, making informed decisions about purchases (like a house), and the relevance of statistics across numerous professional fields, including economics, business, psychology, education, biology, law, computer science, police science, and early childhood development. The ability to effectively analyze and interpret data is presented as a crucial life skill, enhancing confidence in decision-making. A simple example is introduced to illustrate the concept: analyzing the average sleep time of a group using a dot plot, which is a basic form of data visualization.
2. Data Types and Measurement Scales
This section delves into different types of data and measurement scales. It differentiates between nominal, ordinal, interval, and ratio scales, explaining their properties and limitations. Nominal data, such as smartphone brands (Sony, Motorola, Nokia, Samsung, Apple), are categorical with no inherent order. Ordinal data have a defined order but the differences between values are not meaningful. Interval data have a defined order and meaningful differences, but lack a true zero point (e.g., Celsius and Fahrenheit temperature scales). Ratio data have a true zero point, allowing meaningful ratios to be calculated (e.g., exam scores). This discussion highlights how the choice of measurement scale impacts the type of analysis that can be performed on the data. The section clarifies that some calculations are appropriate only for certain data types.
3. Graphical Data Representation Histograms and Other Methods
This section emphasizes the use of graphs for data summarization and organization, stating that statisticians often start by visualizing the data. It mentions several types of graphs: dot plots, bar charts, histograms, stem-and-leaf plots, frequency polygons, and boxplots. The primary focus is on histograms, particularly for larger datasets (100 values or more). The section provides instructions for constructing histograms, including determining the number of bars (intervals or classes), selecting an appropriate starting point for the first interval, and ensuring contiguous boxes. The vertical axis represents either frequency or relative frequency, resulting in the same overall shape. Histograms are highlighted for their ability to show the shape, center, and spread of data.
4. Measuring the Center of Data Mean and Median
This part of the chapter focuses on measuring the central tendency of data, introducing the mean (average) and median as the two most common measures. The calculation of the mean is explained, along with the interpretation of the median as a better measure of center in cases with extreme values or outliers. The text notes that the terms 'mean' and 'average' are often used interchangeably in practice. The median's robustness against outliers is highlighted, making it preferable in certain situations. The section clearly explains that visualizing data is always recommended, as it provides additional context beyond the numerical values.
5. Measuring the Spread of Data Standard Deviation and Variance
This subsection explains how to measure the spread or dispersion of data, introducing the standard deviation and variance as key measures. The calculation of standard deviation is demonstrated, explaining the concept of deviations from the mean and why squaring these deviations is necessary. The text emphasizes the importance of understanding what the standard deviation represents—a measure of how spread out the data are from the mean. It highlights that the standard deviation is more informative for symmetrical distributions but may be less useful for skewed distributions where the first quartile, median, third quartile, minimum, and maximum values provide more insights. The text also clarifies the distinction between sample variance (dividing by n-1) and population variance (dividing by n), emphasizing that the sample variance is an estimate of the population variance, and dividing by (n-1) provides a better estimate.
II.Inferential Statistics Making Inferences from Data
This section introduces inferential statistics, using probability to draw conclusions about a population based on a sample. Key concepts include sampling distributions, sampling variability, and the importance of appropriate sample size. The need for good data collection procedures to ensure reliable inference is highlighted. The document introduces the central limit theorem, showing how sample means form their own normal distribution, and touches upon the law of large numbers.
1. The Importance of Sampling
This section emphasizes the practical necessity of sampling in statistical analysis. It highlights that examining entire populations is often too time-consuming and expensive. Examples illustrate the use of sampling in various contexts: calculating the overall grade point average at a school by sampling students; presidential election opinion polls sampling 1,000 to 2,000 people to represent the entire country; and manufacturers sampling canned drinks to ensure proper fill levels. The inherent variability between samples is highlighted, stating that even with the same sampling method, different samples will likely yield different results. The text stresses that this variability is significant, and larger sample sizes generally lead to results closer to the actual population average, although differences will still likely exist between samples.
2. Understanding Variables and Data Types
This section introduces the concept of a variable (represented by capital letters like X and Y) as a characteristic of interest in a population. It distinguishes between numerical variables (with equal units, such as weight or time) and categorical variables (placing individuals into categories, like political affiliation). An example uses a math student's points earned (numerical) and a person's party affiliation (categorical) to illustrate the difference. The section further distinguishes between quantitative discrete data (from counting, like the number of phone calls) and quantitative continuous data (from measuring, like the weight of backpacks). The importance of recognizing data types is implied as it lays the foundation for the appropriate statistical methods to be applied.
3. The Central Limit Theorem and the Law of Large Numbers
This section introduces the Central Limit Theorem, explaining that as sample size increases, the distribution of sample means approaches a normal distribution, regardless of the shape of the original population distribution. The mean of this sampling distribution is the same as the population mean, and the variance is the original variance divided by the sample size. The section also introduces the Law of Large Numbers, stating that as sample size increases, the sample mean gets progressively closer to the population mean. The Central Limit Theorem is presented as an illustration of the Law of Large Numbers. These theorems are fundamental to inferential statistics, justifying the use of normal distributions to make inferences about population means even when the population distribution isn't normal, provided the sample size is sufficiently large.
III.Confidence Intervals Estimating Population Parameters
This section explains how to construct and interpret confidence intervals, providing a range of values likely to contain the true population parameter (e.g., population mean, population proportion). It describes calculating the margin of error and the importance of the confidence level (e.g., 90%, 95%, 99%). The concept of a point estimate as a single best guess is introduced, and the need to use the Student's t-distribution when the population standard deviation is unknown is highlighted.
1. Introduction to Confidence Intervals
This section introduces the concept of confidence intervals as a method for estimating population parameters. It explains that a confidence interval provides a range of values, calculated from sample data, within which the true population parameter is likely to fall. The general form of a confidence interval is presented as (point estimate - error bound, point estimate + error bound), or (x − EBM, x + EBM). The margin of error (EBM) is highlighted as dependent on the confidence level and sample size. The confidence level is clarified as the percentage of confidence intervals that would contain the true population parameter if the process were repeated many times. The choice of a confidence level of 90% or higher is suggested for greater certainty in conclusions. Examples of point estimates are provided: the average of several rents from a newspaper to estimate the mean rent of two-bedroom apartments, and calculating the percentage of successful basketball shots to estimate the true proportion of successful shots.
2. Calculating Confidence Intervals Using the Standard Normal Distribution
This section discusses the process of calculating confidence intervals, specifically when the population standard deviation (σ) is known. In such cases, a standard normal distribution (Z ~ N(0,1)) is used to calculate the error bound (EBM). The method involves finding the z-score that corresponds to the desired confidence level, essentially identifying the area in the middle of the standard normal distribution representing the specified confidence. This z-score is then used in conjunction with the standard deviation and sample size to compute the error bound. The section introduces the use of z-scores to calculate the confidence interval, linking this concept to the area under the standard normal curve.
3. The Student s t Distribution and Confidence Intervals
This section introduces the Student's t-distribution, explaining its origin and application in calculating confidence intervals when the population standard deviation (σ) is unknown. The explanation notes that using the sample standard deviation (s) directly in place of σ can lead to inaccurate results, particularly with small sample sizes. This section highlights William Gossett's work at the Guinness brewery and how his discovery of the t-distribution addressed this issue. The shape of the t-distribution is described as dependent on the 'degrees of freedom' (n-1), and it approaches the standard normal distribution as degrees of freedom increase. The assumptions required for using the t-distribution are detailed: the underlying population of individual observations should be normally distributed with unknown population mean (µ) and standard deviation (σ); the size of the population is usually irrelevant unless very small; and random sampling is assumed, which is distinct from the normality assumption. The use of t-tables to find t-scores for specified confidence levels and degrees of freedom is described, emphasizing that some tables are formatted to show confidence level in column headings while others might show only tail areas.
4. Confidence Intervals for Population Proportions
This section focuses on calculating confidence intervals for population proportions, relevant when dealing with binomial distributions (e.g., the proportion of voters favoring a candidate). The approach is analogous to that for the population mean, but with different formulas. The section highlights how to identify a proportion problem (absence of mean/average) and introduces the binomial random variable X ~ B(n, p) where n is the number of trials and p is the probability of success. The sample proportion (p') is used for estimation, and for calculating the sample size (n), the estimated proportion is assumed to be 0.5, maximizing the product p'q' (where q' = 1-p'), ensuring a sufficiently large sample. This is done to ensure the confidence interval is within a specified margin of error of the true population proportion.
IV.Hypothesis Testing Making Decisions about Population Parameters
This section covers hypothesis testing, a process for making decisions about population parameters based on sample data. Key concepts include the null hypothesis, alternative hypothesis, calculation of the p-value, and determining statistical significance. The section emphasizes how to interpret results to support or reject hypotheses, determining if observed results are likely due to chance or reflect a real effect. The use of the p-value to judge the strength of evidence against the null hypothesis is highlighted.
1. Introduction to Hypothesis Testing
This section introduces hypothesis testing as a statistical inference method used to make decisions about population parameters based on sample data. It provides examples of claims that might be tested statistically, such as a car dealer's claim about fuel efficiency, a tutoring service's success rate, or a company's claim about women managers' salaries. The section positions hypothesis testing as a crucial tool for statisticians to analyze claims and make informed decisions about population characteristics. The overall goal is to determine if observed data provide enough evidence to reject a null hypothesis, which represents a statement of no effect or no difference.
2. Interpreting p values in Hypothesis Testing
This section illustrates the interpretation of p-values within the context of hypothesis testing. The example uses a baker's claim about the average height of bread loaves. The p-value is introduced as the probability of observing a result as extreme as, or more extreme than, the one obtained if the null hypothesis were true. A small p-value indicates that the observed result is unlikely under the null hypothesis. The example shows a p-value of approximately 0, leading to the conclusion that the evidence strongly contradicts the null hypothesis (that the mean height is at most 15 cm). The interpretation is that a result as extreme as 17 cm is highly improbable if the population mean were actually 15 cm, strongly suggesting the null hypothesis should be rejected in favor of the alternative hypothesis (the mean height is greater than 15 cm). The concept of using the p-value to assess whether results are due to chance alone or a real effect is central to this explanation.
V.Correlation and Regression Exploring Relationships between Variables
This section briefly discusses correlation and regression analysis, methods for exploring relationships between two variables. It emphasizes understanding the assumptions needed for valid correlation analysis and the limitations of extrapolating beyond the observed range of data when making predictions using regression models. The concept of the correlation coefficient is introduced as a measure of the strength and direction of a linear relationship between two variables.
1. Correlation Measuring the Strength of a Linear Relationship
This section introduces correlation as a method to assess the linear relationship between two variables. The text highlights that the analysis is performed on a sample of data points drawn from a larger population, with the goal of inferring whether a similar linear relationship exists within the population. The importance of understanding the assumptions underlying significance testing of the correlation coefficient is mentioned: the data should be viewed as a sample from a larger population, and the analysis seeks to determine if the observed linear relationship in the sample is strong enough to suggest a similar relationship in the population. It emphasizes the limitations of extrapolating beyond the observed range of the data; attempting to predict values outside this range can be unreliable. A practical example involving m-commerce usage over time is briefly introduced to illustrate the idea of visually fitting a line to data and deriving an equation to represent this relationship, acknowledging that different individuals may obtain slightly different equations based on their visual estimations.
2. Linear Regression Predicting Values Based on Relationships
Although not explicitly detailed, the section hints at the connection between correlation and linear regression. The example of visually fitting a line to the m-commerce data implicitly suggests the use of a linear regression model. The process described—plotting points, fitting a line 'by eye,' and using two points to determine the slope and y-intercept—implies a rudimentary linear regression approach. The text mentions that different individuals would likely arrive at slightly different equations, reflecting the subjective nature of this visual fitting process. It indirectly emphasizes that more formal techniques beyond visual estimation are needed for a rigorous analysis of the relationship and precise predictions.
VI.Probability Distributions Understanding Data Variability
This section touches upon different probability distributions, including the normal distribution, Student's t-distribution, binomial distribution, and exponential distribution. Understanding these distributions is crucial for many statistical procedures, particularly in inferential statistics and hypothesis testing. The document highlights the properties of these distributions, especially the need to understand their shapes and parameters for accurate data analysis.
1. The Normal Distribution
The section introduces the normal distribution, describing its symmetrical shape centered around the mean (µ). It explains that the mean and standard deviation (σ) completely define the normal distribution. A change in the standard deviation alters the shape (making it wider or narrower), while a change in the mean shifts the curve left or right. The existence of infinitely many normal distributions is noted, each defined by a specific mean and standard deviation. A special case, the standard normal distribution, is mentioned. The importance of the normal distribution is strongly implied, as it underpins many statistical methods and is a key concept for understanding data variability. The total area under the curve is equal to 1.
2. Other Important Distributions
Beyond the normal distribution, the text mentions other important probability distributions, including the Student's t-distribution and the exponential distribution. The Student's t-distribution is described as having a shape that depends on the degrees of freedom, becoming increasingly similar to the normal distribution as the degrees of freedom increase. Its relevance to confidence intervals when the population standard deviation is unknown is hinted at. The exponential distribution is briefly introduced as a continuous random variable useful for modeling intervals of time between random events, such as the time between emergency arrivals at a hospital. The mean and standard deviation are given for the exponential distribution, along with its probability density function and cumulative distribution function. The inclusion of these distributions points toward the importance of selecting the appropriate distribution for a given situation when conducting statistical analyses.
Document reference
- Principles of Business Statistics (Mihai Nica (Collection Editor), Susan Dean, Barbara Illowsky)
- Sampling and Data: Introduction (Susan Dean, Barbara Illowsky)
- Sampling and Data: Statistics (Susan Dean, Barbara Illowsky)
- Sampling and Data: Key Terms (Susan Dean, Barbara Illowsky)
- Sampling and Data: Data (Susan Dean, Barbara Illowsky)