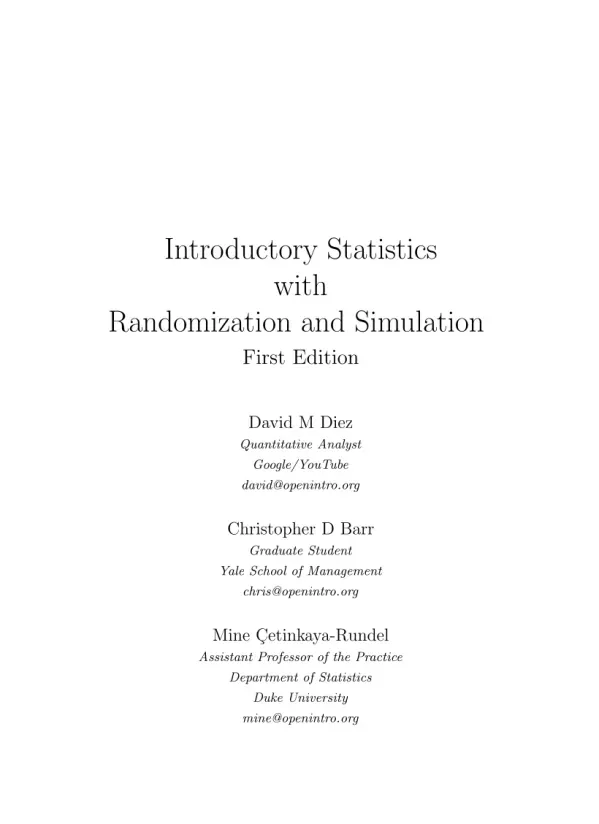
Introductory Statistics with Randomization and Simulation
Document information
Author | David M Diez |
School | Duke University |
Major | Statistics |
Company | Google/YouTube |
Document type | textbook |
Language | English |
Number of pages | 354 |
Format | |
Size | 10.42 MB |
- Statistics
- Data Analysis
- Regression
Summary
I. Introduction to Data
The initial section of the textbook introduces the fundamental concepts of data. It emphasizes the importance of understanding various data structures and their applications in statistical analysis. The authors present a comprehensive overview of data collection principles, which are crucial for gathering accurate and reliable information. Observational studies and sampling strategies are discussed, highlighting their significance in ensuring the validity of statistical inferences. The section also covers the examination of both numerical and categorical data, providing readers with essential tools for data analysis. Notably, the authors state, 'Statistics is an applied field with a wide range of practical applications,' underscoring the relevance of these concepts in real-world scenarios. This foundational knowledge sets the stage for more advanced topics in the subsequent chapters.
1.1 Case Study
The case study section illustrates the practical application of statistical concepts through real-world examples. By analyzing specific cases, readers gain insights into how randomization and simulation techniques can be employed to address complex statistical questions. The authors emphasize the role of case studies in enhancing understanding, stating, 'Case studies are used to introduce the ideas of statistical inference with randomization and simulations.' This approach not only reinforces theoretical knowledge but also demonstrates the practical implications of statistical methods in various fields.
1.2 Data Basics
In this subsection, the authors delve into the essential components of data basics. They discuss the significance of variables, summaries, and graphics in presenting data effectively. The importance of clear data visualization is highlighted, as it aids in interpreting complex datasets. The authors assert that 'data are messy, and statistical tools are imperfect,' which emphasizes the necessity of robust data management techniques. This section serves as a crucial primer for readers, equipping them with the foundational skills needed for effective data analysis.
II. Foundation for Inference
The second section focuses on the foundation for inference, introducing key concepts such as hypothesis testing and the Central Limit Theorem. The authors provide a thorough explanation of randomization case studies, including gender discrimination and opportunity cost, illustrating how these concepts can be applied to real-world problems. The significance of hypothesis testing is emphasized, as it allows researchers to make informed decisions based on sample data. The authors note, 'Understanding the strengths and weaknesses of these tools enables one to learn interesting things about the world.' This section is vital for readers seeking to grasp the principles of statistical inference and its applications in various domains.
2.1 Randomization Case Study Gender Discrimination
This case study examines the implications of gender discrimination through the lens of statistical analysis. By employing randomization techniques, the authors demonstrate how to assess the impact of gender on various outcomes. The analysis reveals the potential biases present in observational data and highlights the importance of rigorous statistical methods in drawing valid conclusions. The authors emphasize that 'randomization is a powerful tool for eliminating confounding variables,' showcasing its effectiveness in producing reliable results.
2.2 Hypothesis Testing
The hypothesis testing subsection provides a detailed exploration of the process involved in testing statistical hypotheses. The authors outline the steps necessary for conducting hypothesis tests, including formulating null and alternative hypotheses, selecting appropriate significance levels, and interpreting results. They stress the importance of understanding Type I and Type II errors, as these concepts are critical for evaluating the reliability of statistical conclusions. The authors assert, 'Hypothesis testing is a cornerstone of statistical inference,' underscoring its relevance in research and decision-making.
III. Inference for Categorical Data
This section addresses the methods used for making inferences about categorical data. The authors discuss various techniques, including the use of the chi-square distribution for testing goodness of fit and independence in two-way tables. The significance of these methods is highlighted, as they allow researchers to draw conclusions about population proportions based on sample data. The authors state, 'Inference for proportions using the normal and chi-square distributions is essential for understanding categorical data,' emphasizing the practical applications of these techniques in fields such as social sciences and market research.
3.1 Inference for a Single Proportion
In this subsection, the authors explore the methods for making inferences about a single proportion. They provide a step-by-step guide on how to conduct tests and construct confidence intervals for proportions. The authors highlight the importance of sample size and variability in determining the accuracy of estimates. They note, 'Understanding the nuances of proportion inference is crucial for effective data analysis,' reinforcing the practical implications of these techniques in real-world scenarios.
3.2 Testing for Independence
The testing for independence subsection focuses on the application of chi-square tests to assess the relationship between two categorical variables. The authors explain the process of constructing contingency tables and calculating expected frequencies. They emphasize the importance of understanding the assumptions underlying chi-square tests, stating, 'Proper application of these tests is essential for valid conclusions.' This section equips readers with the necessary skills to analyze categorical data effectively.
Document reference
- Introductory Statistics with Randomization and Simulation (David M Diez)
- Introductory Statistics with Randomization and Simulation (Christopher D Barr)
- Introductory Statistics with Randomization and Simulation (Mine C ¸ etinkaya-Rundel)
- OpenIntro Statistics
- Creative Commons License