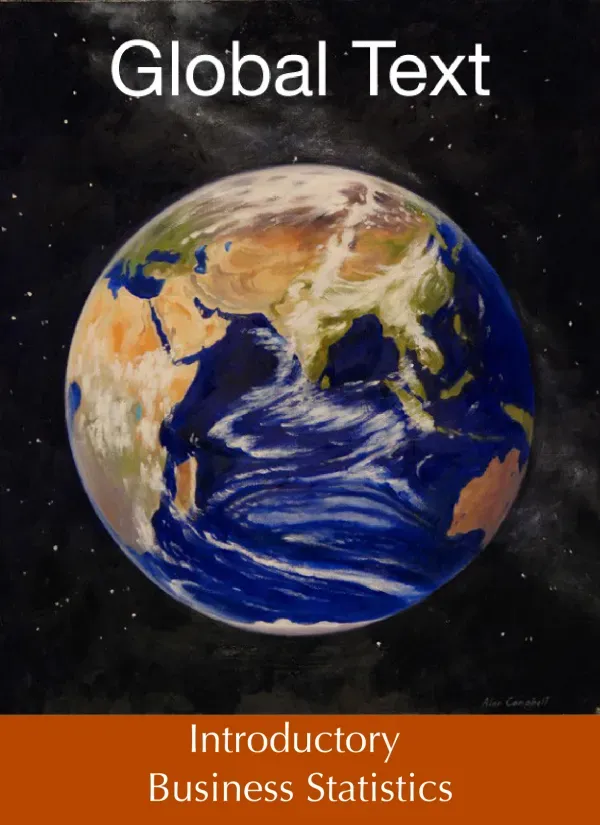
Business Statistics: An Intro
Document information
Author | Thomas K. Tiemann |
instructor/editor | Marisa Drexel |
school/university | Elon University |
subject/major | Business Statistics, Economics |
Document type | Textbook |
Language | English |
Format | |
Size | 2.17 MB |
Summary
I.Estimation and Sampling in Statistics
This section introduces fundamental concepts in inferential statistics, focusing on estimation techniques. It differentiates between point estimates (single-value guesses) and interval estimates (ranges likely containing the true value). The importance of proper sampling is emphasized to ensure accurate inferences about the population from a smaller sample. The text highlights how sample means can estimate population means, but acknowledges that a sample may overestimate or underestimate depending on its composition. The goal is to select representative samples to minimize error and improve accuracy of statistical inferences.
1. Introduction to Estimation
The section begins by defining estimation as a core technique in inferential statistics. The fundamental goal is to draw inferences about a population using data from a sample. Two key types of estimates are introduced: point estimates, which involve selecting a single number as the best guess for a population characteristic, and interval estimates, which provide a range within which the true population characteristic is likely to fall. While point estimates rarely pinpoint the exact value, accurate estimation ensures that the guess isn't far off. The text emphasizes that correctly generating these estimates is a critical skill in statistics, highlighting the need for careful consideration and understanding of statistical methodologies. The challenge lies in balancing the desire for certainty (knowing the exact values for the whole population) against the practical constraints of collecting data for the entire population, as such an endeavor is typically costly and often impossible. The core argument centers on the financial and practical benefits of using samples to infer population characteristics: significant time and resources can be saved, making statistical inference invaluable, particularly in contexts where complete data collection is infeasible. The approach taken in the text aims to minimize the initial cost of learning statistics, maximizing the return on investment by reducing the learning curve and improving the overall efficiency of the learning process. This is analogous to enhancing the Net Present Value (NPV) of an investment by lowering its initial cost.
2. Point Estimates and Sample Means
The explanation proceeds to discuss how sample means are used to infer population means. It illustrates that if a sample includes disproportionately high or low values from the population, the sample mean will not accurately represent the true population mean. However, a sample including a balance of high, low, and middle values will result in a sample mean that is closer to the true population mean, providing a good estimate. The key to effective sampling lies in avoiding skewed samples that overrepresent extreme values. The importance of careful sampling is underscored; the goal is to obtain a sample that is representative of the population. Such a sample would contain a relatively equal proportion of observations across different ranges of values, yielding a sample mean close to the true population mean. The text uses the analogy of a balance between high, low, and middle values to create a clear picture of how this principle relates to getting accurate results. While the sample mean is unlikely to be exactly equal to the population mean, by selecting a representative sample, statisticians can increase their confidence that the sample mean will accurately reflect the population mean and will significantly decrease the margin of error. This careful selection is crucial to statistical inference, as it directly impacts the accuracy and reliability of estimations.
3. Interval Estimation and Confidence Levels
This subsection transitions into a discussion of interval estimation. Instead of relying solely on a single-value point estimate, statisticians use interval estimates to provide a range of values likely to contain the true population characteristic with a specific level of confidence. This approach acknowledges the inherent uncertainty associated with sample data and provides a more nuanced and reliable estimate. The example of estimating the mean age at hiring at Foothill Hosiery illustrates how the process works in practice. Kevin, with a sample of 30 employees, calculates the sample mean and standard deviation and then uses a t-table to determine the interval likely to contain the true population mean with 95% confidence. Ann's approach focuses on the proportion of new hires older than 35. She chooses a sample of 100 personnel files, and uses the normal distribution to create a 95% confidence interval for the population proportion. These examples demonstrate how confidence intervals use sampling distributions to link sample statistics with population parameters. The method is illustrated by two distinct examples: Kevin’s estimation of mean age at hire, which is done with 95% confidence, and Ann's estimation of the proportion of employees over 35, done also with 95% confidence. In Kevin's case, a sample of 30 employees resulted in a sample mean and standard deviation, which were then used to estimate an interval that contains the true population mean with a probability of 0.95. Ann's method focuses on the proportion of new hires older than 35, using a sample of 100 files and 95% confidence.
II.Univariate and Multivariate Statistics
This section distinguishes between univariate statistics, which analyze single variables (e.g., average shoe size), and multivariate statistics, which examine relationships between multiple variables (e.g., correlation between GPA and shoe size). Multivariate statistics are powerful for prediction. The concept of an observation is introduced, representing a data point for each member of a group. The discussion includes the visualization of data using different graph types, appropriate for both discrete and continuous variables. The key is understanding that for continuous variables, the area under a curve represents the relative frequency of observations within a given range.
1. Univariate vs. Multivariate Statistics
The core distinction in this section lies between univariate and multivariate statistics. Univariate statistics focus on analyzing single variables within a population, aiming to understand the characteristics of that single variable. An example provided is determining the average shoe size of business students. This involves collecting data on shoe sizes and calculating descriptive statistics like the mean and standard deviation to understand the central tendency and dispersion of shoe sizes within the student population. This approach provides insights into the distribution of a single characteristic, making it valuable for understanding the nature of a specific variable in isolation. In contrast, multivariate statistics delve into the relationships between two or more variables within a population. The text illustrates this with the question of whether students with high GPAs tend to have larger feet. This requires collecting data on both GPA and foot size, exploring their correlation to understand if higher GPAs are associated with larger feet. This involves more complex statistical techniques like correlation analysis or regression analysis. The key difference is that multivariate statistics are used for prediction and are significantly more powerful for identifying trends and causal relationships.
2. The Concept of Observation
The concept of an observation is introduced as a crucial element in both univariate and multivariate analysis. An observation represents a single data point collected for each member of the group under study. Whether the population involves a single characteristic (univariate) or multiple characteristics (multivariate), each member contributes one observation. For instance, in the example of determining the average shoe size of business students, each student's shoe size constitutes a single observation. In the multivariate example of exploring the relationship between GPA and foot size, each student's data (GPA and foot size) would form a single observation containing two data points. This concept is fundamental to data organization and analysis, providing a standardized unit for both descriptive and inferential statistical methods. Understanding observations allows us to build a systematic approach to data analysis, making sure we can properly organize and process the relevant information to conduct any statistical calculations or modelling that we wish to perform.
3. Data Visualization Discrete vs. Continuous Variables
The section further emphasizes the importance of data visualization and how it differs depending on the nature of the variables. It highlights the distinction between discrete and continuous variables, emphasizing how these distinctions affect data representation. Discrete variables, like sock sizes, take on only a limited number of values; therefore, bar graphs are typically sufficient to illustrate their frequency distribution. Continuous variables, like student weight, can take on an infinite number of values (even if typically rounded). The text argues that a 'connect-the-dots' graph is better suited to illustrate the distribution of a continuous variable since the relative frequency of any single value is minimal. The relative frequency instead resides within a range of values; hence, the area under the curve becomes the relevant feature of this type of graph, the area under the graph between two values representing the proportion of observations falling within that particular range. This discussion is crucial to help statisticians visualize their data in a manner that accurately reflects the distribution and ensures that they can properly interpret their data.
III.Understanding Skewness and Distributions
This section describes measures of skewness in a data distribution, explaining how cubing deviations from the mean (in contrast to squaring) provides information on the direction and magnitude of the skew. A negative skew indicates a long tail to the left and a positive skew a long tail to the right. This lays groundwork for understanding how data distributions, especially their shape, influence statistical hypothesis testing.
1. Measuring Skewness using Cubing
This section focuses on understanding and measuring skewness in data distributions. It explains that while squaring the deviations from the mean always results in positive numbers, cubing them retains the sign (positive or negative). This is significant because cubing also magnifies the impact of extreme values much more than squaring. The text illustrates this with the example of a distribution with a long tail to the left. In such a distribution, there are a few observations significantly smaller than the mean, resulting in large negative deviations. When these deviations are cubed, they create very large negative numbers. Because there are far fewer large positive deviations, the sum of the cubed deviations will be negative, indicating a negative skew. Conversely, a long tail to the right (positive skew) would result in a positive sum of cubed deviations. The explanation emphasizes that for a perfectly symmetric distribution, the measure of skew will be zero, as the negative and positive cubed deviations will balance each other out. This understanding of skew is crucial in descriptive statistics, providing valuable information about the shape of the data distribution and indicating potential outliers or unusual patterns within the data.
IV.Normal and t Distributions
This section introduces the normal distribution (the bell curve) and the t-distribution. The central limit theorem is discussed, explaining the relationship between the sampling distribution of means and the original population. It highlights that the variance of the sampling distribution is related to the population variance and the sample size (n). Larger samples lead to smaller variances in the sampling distribution, making inferences about the population mean more precise. The t-distribution is particularly important when the population variance is unknown, allowing for the estimation of the population mean and variance using sample data.
1. Introduction to the Normal Distribution
The section introduces the normal distribution, also known as the bell-shaped curve, highlighting its prevalence in describing various naturally occurring, manufactured, and human performance outcomes. It is termed 'normal' due to its frequent appearance in these contexts. An example given is grading on a 'bell curve,' where a teacher tries to fit student grades into a normal distribution, a practice considered valid for large classes with objective assessments. The normal distribution's characteristic shape, with most values concentrated around the mean and fewer values at the extremes, is described. This symmetrical nature of the distribution is significant in probability and inferential statistics. The importance of the normal distribution in statistics is emphasized, citing its use in describing many real-world phenomena. This introductory discussion of the normal distribution sets the stage for understanding its role in statistical inference. The text particularly points out the practical applications of the normal distribution, and how it is relevant to different situations that statisticians might encounter.
2. The t Distribution and the Central Limit Theorem
The section then introduces the t-distribution, a common sampling distribution used in statistical inference. The t-distribution is constructed by repeatedly taking samples of the same size from a normal population, calculating a t-statistic for each sample, and creating a relative frequency distribution of these t-statistics. The resulting distribution, known as the t-distribution, has properties that make it useful for making a variety of inferences. The central limit theorem is then described as providing crucial knowledge regarding the relationship between sample means and population means. This theorem helps statisticians make inferences about the population mean, a key parameter in many statistical analyses. The t-distribution's flexibility is emphasized – its application extends to various situations and sample statistics, making it one of the most important tools for statisticians in bridging the gap between sample data and population parameters. The text highlights how the standard deviation of the sampling distribution shrinks as the sample size grows. This is intuitively explained: as sample sizes increase, it becomes less likely that a sample's mean will deviate substantially from the population's true mean. The relationship between the variance of the sampling distribution and that of the original population, is also highlighted.
3. The t Distribution and Population Variance
The discussion then shifts to the practical application of the t-distribution, particularly when the population variance is unknown. This is often the case in real-world scenarios, unlike the idealized situations presented in textbooks. Statisticians use the sample variance as an unbiased estimator of the population variance, recognizing that the sample variance is unlikely to be exactly equal to the true population variance, but, on average, it will equal the true value. The text emphasizes the importance of this estimation technique, as it allows statisticians to make inferences about the population mean even when the population variance is not known. The role of the t-distribution is further detailed; by incorporating the sample variance, it allows statisticians to create more realistic and reliable estimates for the population mean and variance. The text also mentions that the t-distribution is symmetric, mirroring the symmetry of the sampling distribution of means when the original population is normal. This symmetry is significant because it simplifies the calculation and interpretation of statistical tests based on the t-distribution.
V.Making Estimates Means Proportions and Variances
This section details how to make interval estimates for different population characteristics. It covers methods for estimating the population mean (using the t-distribution), the population proportion (using the normal distribution), and the population variance (using the chi-squared distribution). Each method involves a similar process: choosing a confidence level (e.g., 95%), collecting sample data, calculating relevant statistics, and using the appropriate sampling distribution to create a confidence interval.
1. The Basic Approach to Making Inferences
The section starts by establishing that the most fundamental inference about a population is estimating the location or shape of its distribution. It explains that the sample mean serves as an unbiased estimator of the population mean, though it's rarely exactly accurate. Statisticians prefer to estimate an interval that very likely contains the true population mean, a process called interval estimation. The text outlines a general approach to making inferences from samples: First, choose the population characteristic of interest. Second, select a sample and calculate the relevant sample statistic. Then, utilize the appropriate sampling distribution to link the sample data to the population. Finally, construct a confidence interval around the sample statistic to estimate the population characteristic. This section emphasizes a structured approach to drawing inferences, highlighting that the methods are analogous regardless of whether you are making inferences about the mean, proportion, or variance. This provides a common framework for different types of inferences. The overall aim is to provide a robust and statistically sound methodology for drawing conclusions about a population using data obtained from a sample.
2. Estimating the Population Mean
This subsection illustrates interval estimation using the example of Foothill Hosiery's hiring practices. John McGrath suspects age discrimination, and Kevin aims to estimate the average age of hired workers. With over 2500 employees in the last fifteen years, collecting data for all would be costly. Therefore, Kevin chooses a sample of 30 employee files and calculates the sample mean and standard deviation. The sample mean age at hiring is found to be 24.71 years, with a standard deviation of 2.13 years. He uses the t-distribution with 29 degrees of freedom (df) and the t-table to find that 95% of t-scores are between ±2.045, generating an interval estimate with 95% confidence. This concrete example shows how to combine sampling, calculation, and a sampling distribution to generate a confidence interval for the population mean. The selection of a 95% confidence level shows the application of established statistical practices. The use of the t-distribution accounts for the fact that the population standard deviation is unknown, demonstrating the importance of the appropriate choice of the sampling distribution.
3. Estimating Population Proportion and Variance
The next part extends the concept to estimating population proportions. Ann, also at Foothill Hosiery, aims to determine the proportion of new hires aged 35 or older. Again, surveying all 2500+ employees is impractical, so she selects a sample of 100 files (with replacement). She observes 17 employees aged 35 or older in her sample and uses the normal distribution to construct a 95% confidence interval, finding that 95% of z-scores lie between ±1.96. This demonstrates the application of interval estimation to proportions. The use of the normal distribution highlights the link between sampling distributions and the specific type of estimate. The choice of 95% confidence again shows the importance of choosing an appropriate confidence level. Finally, the section touches upon estimating population variance, a crucial element in quality control. Kevin, tasked with assessing the variance in sock weight at Foothill Hosiery, uses his existing data and chooses a 90% confidence level to make an interval estimate. This shows the use of the chi-squared distribution for the estimation of variance.
VI.Hypothesis Testing The Basic Strategy
This section outlines the general strategy for hypothesis testing. It involves formulating a null hypothesis (the default assumption) and an alternative hypothesis (what you are testing). A significance level (alpha, α) is chosen to determine the threshold for rejecting the null hypothesis. The process uses sampling distributions (like the t-distribution or normal distribution) to evaluate whether sample data provides enough evidence to reject the null hypothesis in favor of the alternative.
1. Formulating Hypotheses
The section begins by explaining the fundamental structure of hypothesis testing. This involves formulating two contrasting statements about the population: the null hypothesis and the alternative hypothesis. The null hypothesis represents the default assumption, essentially stating that the population is 'like this,' meaning it's no different from the usual or expected state. The alternative hypothesis posits that the population is 'like something else,' indicating a difference from the norm. These two hypotheses must collectively encompass all possible outcomes. The text emphasizes that the hypotheses are statements about the population parameters (means, proportions, or distributions) and not directly about the sample data. To clarify the process, the text offers an informal interpretation: The null hypothesis equates to 'I am almost positive that the sample came from a population like this,' while the alternative hypothesis translates to 'I really doubt that the sample came from a population like this, so it probably came from a population that is like something else'. This informal translation better reflects the inherent uncertainty involved in statistical inference, acknowledging that we can never be 100% certain about our conclusions. The overall message is that the process of hypothesis testing is fundamentally about comparing observed sample data against an expectation described by a null hypothesis.
2. The Decision Rule and Sampling Distributions
The core of hypothesis testing revolves around the decision rule, which guides whether to accept or reject the null hypothesis. This decision is made based on how far the sample statistic deviates from the null hypothesis value, considering the variability inherent in sampling. The decision rule is typically expressed in terms of standardized sampling distributions (t-distribution, normal z-distribution, etc.), providing a link between the sample statistic and the population under consideration. The text highlights that the use of these standardized distributions is what enables comparison between sample data and population parameters. If the sample statistic falls within the region determined by the decision rule as being unlikely under the null hypothesis, then the null hypothesis is rejected. The section strongly advises against simply memorizing formulas for different statistical tests. Instead, it recommends understanding the underlying logic—recognizing that various hypothesis tests are variations on a fundamental theme, involving comparing a sample statistic against a sampling distribution. This approach allows for a more flexible and robust understanding of hypothesis testing, preventing the need to memorize many individual procedures.
3. Significance Levels and Type I Error
The text discusses the role of the significance level (alpha, α) in hypothesis testing. Alpha defines the probability of rejecting the null hypothesis when it is actually true (Type I error). A common default value is 5%, implying a 5% chance of making a Type I error. The choice of alpha involves a trade-off, balancing the risk of making Type I error against the risk of failing to reject the null hypothesis when it's actually false (Type II error). There's no single ‘best’ alpha; the appropriate value depends on the relative costs associated with each type of error. Many researchers use a 5% threshold by default, demonstrating established practice. However, researchers may choose to report the P-value (the probability of observing the obtained results or more extreme results if the null hypothesis is true) instead of selecting alpha beforehand. The P-value allows the reader to choose their own significance level depending on their acceptable risk tolerance. This modern approach is seen as providing more transparency and flexibility than a predetermined alpha, promoting a more informed and data-driven interpretation of statistical results.
VII.Specific Hypothesis Tests t tests and Goodness of Fit
This section explains different types of t-tests: one-sample t-test (comparing a sample mean to a hypothesized population mean), two-sample t-test (comparing means of two independent samples), and paired samples t-test (comparing means of paired observations). It also introduces the chi-squared (χ²) test for determining if a sample distribution fits a hypothesized population distribution (goodness-of-fit test). These tests allow inferences about population parameters using sample data and specified significance levels (alpha).
1. Different Types of t tests
This section details various applications of t-tests, highlighting their versatility in hypothesis testing. The text describes three main types: the one-sample t-test, used to compare a sample mean to a hypothesized population mean; the two-sample t-test, employed to compare the means of two independent samples; and the paired samples t-test, designed for comparing the means of paired observations (e.g., before-and-after measurements on the same individuals). Each test type addresses a specific research question. The one-sample test is used to assess if a sample comes from a population with a particular mean. The two-sample test determines if two samples originate from populations with the same mean, and the paired-sample test focuses on the equality of means in paired sets. While differing in their application, all t-tests follow a consistent strategy: formulate the null and alternative hypotheses (ensuring the null contains an equals sign), select a significance level (alpha, α), calculate the relevant t-statistic, and then consult the t-table to determine if the sample data supports the null or alternative hypothesis. This consistent approach simplifies the application of this essential statistical tool, allowing researchers to efficiently and accurately test many different types of questions.
2. The Sock Marketing Example A Chi Squared Test
The section then delves into a specific example—Ann's marketing campaign for 'Easy Bounce' socks. The challenge is to determine whether the distribution of sock sizes among volleyball players matches Foothill Hosiery's current production. To address this, the text introduces the chi-squared (χ²) test, a statistical method particularly relevant for testing the goodness of fit between a sample distribution and a hypothesized population distribution. Ann has collected a sample of 97 volleyball players' sock sizes and wants to know if the distribution of sizes is comparable to the existing sock sizes the company produces. This is a goodness-of-fit test. Ann starts by calculating the expected frequencies for each sock size in her sample, based on the company's current production ratios. She compares these expected frequencies to the observed frequencies (from her sample), using these values to calculate a chi-squared statistic. The calculated chi-squared statistic is compared to a critical value from a chi-squared distribution table; if this calculated value exceeds the critical value, then the null hypothesis (that the distributions are the same) is rejected. This demonstrates a practical application of the chi-squared test, showcasing its suitability for addressing questions of distributional comparisons. The importance of carefully selecting a significance level (alpha) to define the threshold for rejecting the null hypothesis is again emphasized.
VIII.F tests and ANOVA
This section covers F-tests, primarily for comparing variances of two or more populations using ANOVA (Analysis of Variance). It explains how to use F-tables to determine if the variances are significantly different. The choice of alpha (α) impacts the decision rule. The section notes the complexities of using F-tables, especially when considering both tails of the distribution.
1. Using F tables to Test for Equal Variances
This section explains how to use F-tests and F-tables to test the hypothesis that two sample variances come from populations with equal variances. The process involves calculating an F-statistic by dividing the larger sample variance by the smaller sample variance. The resulting F-statistic is then compared to a critical value obtained from an F-table. The F-table, however, introduces some complexity: it's usually presented as a one-tailed table, showing the critical F-value that separates the right tail from the bulk of the distribution. Because researchers are usually interested in testing whether one variance is greater than another, this one-tailed approach is often sufficient. However, to test for equal variances, researchers must consider both tails of the F-distribution, which complicates the process. Researchers often simply divide the larger sample variance by the smaller one and use a one-tailed test, effectively treating the problem as a one-sided test even if a two-sided approach would be more accurate. The text emphasizes the importance of selecting a significance level (alpha, α) before consulting the F-table, recognizing that this α determines the probability of incorrectly rejecting the null hypothesis. The choice of which variance goes into the numerator and which goes in the denominator impacts the degrees of freedom used for the F-test, adding another layer of complexity to the method.
2. The Challenges of Using F tables
The section acknowledges the inherent challenges associated with using F-tables, emphasizing their intricacy, especially when dealing with two-tailed tests. Before using the tables, researchers must choose a significance level (alpha, α), which represents the probability of rejecting the null hypothesis when it's true. The usual choice is 5% (α = 0.05). F-tables are typically printed as one-tailed tables, presenting the critical F-value for the upper tail of the distribution. This is because many applications of the F-test focus on whether the variance of one group is significantly greater than the variance of another. Testing for equal variances, however, requires considering both tails of the distribution, which isn't directly addressed in standard one-tailed tables. The process involves determining the degrees of freedom (df) for each sample (n-1), understanding that the larger variance sample is assigned to the column while the smaller variance sample is assigned to the row. The text points out the common practice of simply dividing the larger variance by the smaller variance and consulting the one-tailed F-table as a simplification, noting that this practice effectively reduces the test to a one-sided analysis, which may not be suitable in all circumstances. In more rigorous situations, a two-tailed approach would be necessary. The explanation provides a practical guide on handling F-tables while highlighting the complexities that may arise when testing for the equality of variances between groups, adding further nuances to the already complex aspects of statistical hypothesis testing.
IX.Non parametric Statistics
This section contrasts parametric and non-parametric statistics. Parametric tests require certain assumptions (like normality of the data), while non-parametric tests are used when these assumptions are violated or when data are only ordinal (ranked). The Mann-Whitney U test is presented as a non-parametric alternative for comparing the distributions of two groups, using rank sums instead of means. This method is particularly useful when dealing with ranked data or when assumptions for parametric tests are not met.
1. When to Use Non parametric Statistics
This section introduces non-parametric statistics, explaining their use when the assumptions of parametric tests are not met. Parametric tests, such as t-tests and ANOVA, assume that the data is normally distributed and that the data is measured on an interval or ratio scale. Non-parametric statistics are used when the parameters of the population are not measurable or do not meet these assumptions. This could be because the data is only ordinal (ranked) or the sample size is too small for the central limit theorem to apply. If the data only provides an ordering of observations, without information on the intervals between the observations, then neither the mean nor variance can be reliably computed, necessitating non-parametric methods. Even if the data is measured on an interval or ratio scale, parametric techniques are only appropriate if the underlying population is normally distributed. While large samples can often mitigate deviations from normality, small sample sizes combined with non-normal distributions necessitate the use of non-parametric methods. The text highlights that non-parametric methods are generally less precise than their parametric counterparts, reflecting a tradeoff between the need to meet stringent assumptions and the level of precision achievable in statistical analysis. This emphasizes that there are situations where employing non-parametric tests is simply more appropriate or necessary.
2. The Mann Whitney U Test A Non parametric Example
The section introduces the Mann-Whitney U test as a specific non-parametric method. This test is used to determine if two independent samples likely came from populations with the same distribution. Instead of comparing means (as in a t-test), this test utilizes rank sums. The example involves comparing the rankings of cities in two regions (east and west). The observed rank sums are calculated for both groups. The Mann-Whitney U statistic is then calculated, using a formula based on the rank sums of both groups. A small U-value indicates that the data does not support the null hypothesis (that the groups come from the same distribution). The null hypothesis is that the rank sums of both groups would be similar if the two groups were drawn from the same population. The decision of whether to accept or reject the null hypothesis is made by comparing the calculated U-statistic to a critical value obtained from a Mann-Whitney U table, considering the sample sizes of the two groups. The choice of alpha (α) for the significance level dictates how stringent the criteria are for rejecting the null hypothesis. The text also highlights how to conduct a one-tailed versus a two-tailed test using the Mann-Whitney U statistic. The text emphasizes the use of this test for situations involving ranked data or when assumptions for parametric tests are not met.
X.Correlation and Regression Analysis
This section introduces correlation, measuring the strength and direction of the relationship between two variables. It distinguishes between parametric correlation (requiring interval data from normal populations) and non-parametric correlation (like Spearman's rank correlation coefficient, usable with ranked data). The section then explains regression analysis, a powerful multivariate technique that estimates the functional relationship between variables, allowing predictions and inferences about the population. The text discusses linear regression models, the estimation of slopes and intercepts, and hypothesis testing using t-tests and F-tests to assess the significance of the relationship between variables.
1. Correlation Measuring the Relationship Between Variables
This section introduces the concept of correlation, defining it as a measure of how well two variables are tied together. Perfect correlation exists when a change in one variable always corresponds to a consistent change in the other. Correlation coefficients range from -1 to +1. A positive coefficient indicates that the variables move in the same direction (as one increases, the other tends to increase), while a negative coefficient signifies an inverse relationship (as one increases, the other tends to decrease). The absolute value of the coefficient represents the strength of the relationship: a value close to 1 (positive or negative) denotes a strong relationship, while a value near 0 suggests a weak or nonexistent relationship. The text distinguishes between parametric correlation (requiring interval data and normally distributed populations) and non-parametric approaches like Spearman's rank correlation coefficient, which can be applied to ranked data. The explanation aims to provide a clear understanding of how to interpret correlation coefficients in terms of both the strength and direction of the relationship between two variables. The section also touches on the assumptions behind the calculation and the use of different types of correlation measures depending on the nature of the data.
2. Regression Analysis Estimating Functional Relationships
The section moves on to regression analysis, a powerful multivariate technique for inferring functional relationships between variables. It is described as one of the most used and powerful multivariate techniques available to statisticians. The simplest form of regression is linear regression, where the relationship between variables is expressed as a linear equation (y = α + βx), with α representing the intercept and β the slope. These parameters are estimated using sample data, and the resulting equation allows for predictions of the dependent variable (y) based on values of the independent variable (x). The text explains that even with a strong relationship, the predictions from the regression equation will not be perfect, due to inherent variability. The process of regression analysis involves finding the line of best fit, determining the slope and intercept parameters (α and β). The accuracy of these estimates is influenced by sample size and the overall relationship between the variables. The section also touches upon the possibility of extending this concept to multiple independent variables and non-linear relationships, demonstrating the breadth and power of this fundamental multivariate technique. Hypothesis testing using t- and F-distributions is also mentioned in the context of evaluating the statistical significance of the relationships identified through regression analysis.
3. Regression and Correlation The Connection
The final part of this section discusses the relationship between correlation and regression. It illustrates how a strong correlation will result in a regression line that accurately predicts y-values given x-values. The text explains that when a population exhibits a strong linear relationship between variables (x,y), several aspects align: the covariance between x and y is high, a regression line can be easily constructed and provides accurate predictions, and the correlation coefficient will be close to +1 (or -1). This is because a high correlation implies that the observed data points cluster tightly around a straight line, easily fitted by the regression equation. Conversely, a low correlation would suggest that the points are scattered, making an accurate regression line difficult to establish. This section illustrates the close connection between correlation and regression analysis, demonstrating that strong correlations are necessary (but not sufficient) to ensure that a regression analysis will yield an accurate and reliable prediction equation.
Document reference
- F-tables