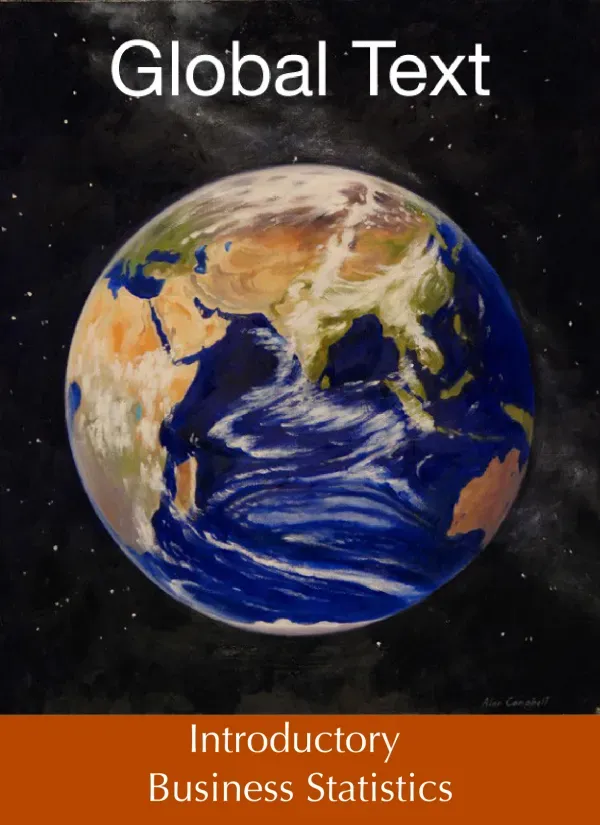
Introductory Business Statistics by Thomas K. Tiemann
Document information
Author | Thomas K. Tiemann |
instructor | Thomas K. Tiemann, Editor-In-Chief |
School | Elon University |
Major | Business Statistics |
Year of publication | 2010 |
Place | North Carolina |
Document type | textbook |
Language | English |
Number of pages | 82 |
Format | |
Size | 2.17 MB |
- Statistics
- Descriptive Statistics
- Inferential Statistics
Summary
I. Introduction to Statistics
Statistics serves as a vital tool in transforming data into information. It encompasses two primary definitions: the first focuses on descriptive statistics, which involves summarizing and organizing data, while the second pertains to inferential statistics, which makes inferences about populations based on sample data. Understanding these definitions is crucial for grasping the broader concepts of statistics. The text introduces two fictional characters, Ann Howard and Kevin Schmidt, who navigate statistical challenges in a business context. Their experiences illustrate the practical applications of statistics in real-world scenarios, emphasizing the importance of statistical literacy in decision-making processes.
1.1 Data vs. Information
The distinction between data and information is fundamental in statistics. Data represents raw numbers, while information is derived from processing and summarizing that data. For instance, a list of test scores is data, but calculating the average score transforms it into information. This section highlights the importance of understanding how to convert data into actionable insights, which is essential for effective problem-solving in business contexts. The example of Foothill Mills illustrates how Ann and Kevin utilize statistical methods to identify new market opportunities, showcasing the practical relevance of statistics in business decision-making.
II. Descriptive Statistics and Frequency Distributions
Descriptive statistics provide a framework for summarizing and interpreting data through various measures such as mean, median, and mode. This section delves into frequency distributions, which organize data into categories, allowing for easier analysis and visualization. The significance of these statistical tools lies in their ability to present complex data in a comprehensible manner. By employing descriptive statistics, businesses can identify trends and patterns that inform strategic decisions. The text emphasizes the role of visual aids, such as graphs and charts, in enhancing the understanding of data distributions, making it easier for stakeholders to grasp key insights.
2.1 Importance of Frequency Distributions
Frequency distributions are essential for analyzing the distribution of data points across different categories. They provide a clear picture of how data is spread, highlighting areas of concentration and potential outliers. This analysis is crucial for businesses seeking to understand customer behavior or market trends. The text illustrates how Ann and Kevin can utilize frequency distributions to assess the performance of their products, enabling them to make informed decisions about marketing strategies and product development.
III. Inferential Statistics and Hypothesis Testing
Inferential statistics extend beyond mere description, allowing for predictions and generalizations about a population based on sample data. This section introduces hypothesis testing, a method used to determine the validity of assumptions made about a population. The process involves formulating a null hypothesis and an alternative hypothesis, followed by statistical testing to draw conclusions. The significance of hypothesis testing lies in its ability to provide a structured approach to decision-making, reducing uncertainty in business environments. The text emphasizes the importance of understanding the underlying principles of hypothesis testing to effectively apply these methods in real-world scenarios.
3.1 The Role of Hypothesis Testing
Hypothesis testing plays a critical role in validating business strategies and decisions. By testing assumptions against sample data, businesses can assess the likelihood of various outcomes. This section discusses the implications of statistical significance and the potential consequences of Type I and Type II errors. Understanding these concepts is vital for making informed decisions that can impact a company's success. The text provides practical examples of how Ann and Kevin can apply hypothesis testing to evaluate marketing campaigns, product launches, and other strategic initiatives.
IV. Regression Analysis
Regression analysis is a powerful statistical tool used to examine relationships between variables. This section explores the fundamentals of regression, including correlation and covariance, and their applications in predicting outcomes. By understanding how different factors influence one another, businesses can make data-driven decisions that enhance performance. The text highlights the importance of regression analysis in identifying key drivers of success, enabling companies to allocate resources effectively and optimize strategies. The practical applications of regression analysis are illustrated through case studies, demonstrating its relevance in various business contexts.
4.1 Practical Applications of Regression
The practical applications of regression analysis are vast, ranging from sales forecasting to market research. This section discusses how businesses can leverage regression models to predict future trends based on historical data. By analyzing relationships between variables, companies can identify opportunities for growth and areas for improvement. The text emphasizes the importance of continuous data analysis in adapting to changing market conditions, ensuring that businesses remain competitive and responsive to customer needs.
Document reference
- Introductory Business Statistics (Thomas K. Tiemann)
- Creative Commons Attribution 3.0 License (Thomas K. Tiemann)
- Jacobs Foundation (Jacobs Foundation)
- Dartmouth College (Dartmouth College)
- Vanderbilt University (Vanderbilt University)