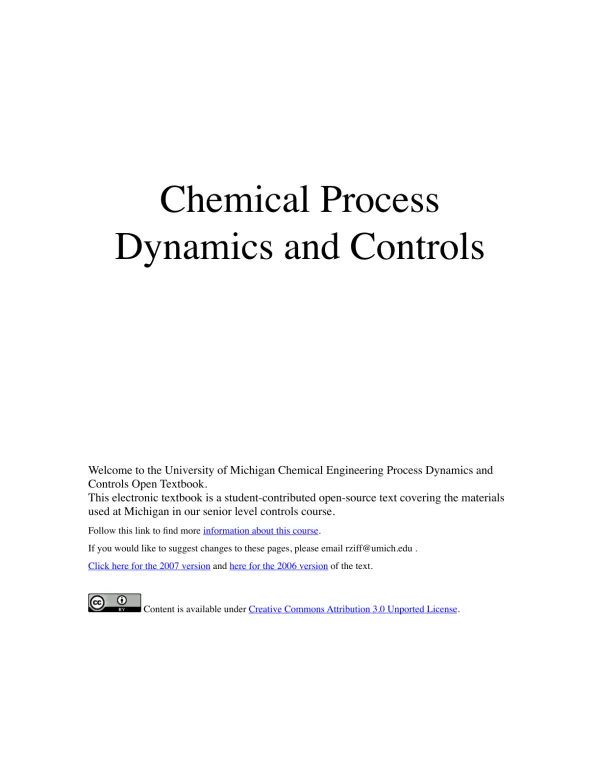
Process Control Open Textbook
Document information
Author | Student Contributors |
instructor/editor | Rziff |
School | University of Michigan |
Major | Chemical Engineering |
Document type | Electronic Textbook |
Year of publication | 2007, 2006 (Multiple Versions Available) |
Language | English |
Format | |
Size | 37.60 MB |
Summary
I.Objectives of Process Control and Level Control Systems
Process control aims to maintain operational conditions and setpoints, preventing deviations that lead to losses. A key example is level control in a tank, where sensors and valves maintain the optimal fluid height, preventing overflow. This is crucial for efficient and safe operation. Failures in such systems, as seen in incidents like the Texas City refinery explosion in 2005 (15 fatalities), highlight the critical role of process control systems and safety systems.
1. Maintaining Process at Operational Conditions and Setpoints
This subsection emphasizes the core goal of process control: maintaining processes at desired operational conditions and setpoints. Deviation from these optimal states can cause substantial losses for a company, impacting budget, yield, safety, and overall quality objectives. The text illustrates this with a scenario of an uncontrolled water tank in a heating and cooling system, where continuous filling without drainage leads to overflowing. This uncontrolled behavior highlights the need for implementing control systems. The importance of maintaining steady-state conditions is stressed, pointing out that various environmental changes (e.g., feed composition, temperature fluctuations, flow rate variations) can also cause a process to stray from these desired conditions. The example serves to underscore the critical need for consistent process monitoring and active intervention to prevent costly and potentially dangerous situations. The implementation of control valves and level sensors provides a solution for the uncontrolled water tank problem, thus ensuring that the process remains stable and within its operational parameters. The concept of instability, where process variables oscillate beyond acceptable limits, is also mentioned as a potential cause for deviation from ideal operating conditions.
2. Level Control The Example of a Fluid Tank
This subsection focuses on a specific type of process control—level control—using a fluid tank as a prime example. The primary concern is managing the height of the fluid within the tank to prevent overflow. This is achieved through the strategic integration of control valves and level sensors. The level sensors continuously monitor the fluid height, providing real-time data to the control system. This data is then used by the system to adjust the control valves, regulating the inflow and outflow of fluid. This ensures that the fluid level remains within the desired range, preventing both overflow and potentially dangerous situations. The introduction of this system transforms the previously uncontrolled tank, prone to overflowing, into a managed and stable system, illustrating the significant impact that effective process control can have on preventing system failures and optimizing operational efficiency. The simple addition of these components demonstrates a basic yet effective approach to process control, ensuring that the fluid level remains within safe and efficient operational parameters.
3. The Impact of Process Control Failures Case Studies
This section underscores the critical importance of robust process control systems by examining real-world instances of catastrophic failures. The discussion centers on two significant incidents: the Three Mile Island (TMI-2) nuclear power plant accident and the Texas City refinery explosion. The TMI-2 accident, while successfully contained, is analyzed to illustrate how control system design flaws hampered the operators' ability to cool the reactor core, leading to a near-meltdown. This near-disaster highlights the potentially devastating consequences that can arise from design failures in complex systems. The Texas City refinery explosion in March 2005 resulted in 15 fatalities and serves as a stark reminder of the severe risks associated with inadequate process safety and operator training. The explosion's root causes are identified as deficiencies in start-up procedures, operator training, and the design of the safety relief system. The events described in both case studies underscore the far-reaching ethical responsibilities of engineers in ensuring the safe and proper operation of industrial processes, extending beyond the confines of a single company and impacting the surrounding community and environment. These accidents emphasize the critical need for meticulous design, stringent safety protocols, and comprehensive operator training in order to mitigate potential risks.
II.Introduction to Distributed Control Systems DCS
Distributed Control Systems (DCS), also known as digital control systems, automate manufacturing processes. DCS allows remote monitoring, process modeling, and optimization for improved safety and profitability, replacing earlier manual and pneumatic control methods. They are the brain of modern industrial process control.
1. DCS The Brain of the Control System
The section introduces Distributed Control Systems (DCS), also known as Digital Control Systems, as the central component of modern process control. DCS is described as the "brain" of the control system, primarily used for automating manufacturing processes and managing the logic of major unit operations. The evolution of DCS is briefly traced, contrasting it with the earlier reliance on pneumatic devices and manual valve operation. A key advantage highlighted is the ability to model systems, recording and managing processes conveniently from a computer screen. This capability enables remote process control, facilitating a deeper understanding of process operations and paving the way for improvements geared towards increasing both safety and profit margins. This advancement allows for significant improvements in operational efficiency, leading to increased profitability and a reduction in the risk of costly and potentially dangerous accidents.
2. Advantages and Impact of DCS
This part of the section elaborates on the significant advantages offered by DCS. The ability to monitor and manage processes remotely is a major benefit, surpassing the limitations of older pneumatic systems and manual control methods. Crucially, DCS facilitates process modeling, offering valuable insights into how processes function and providing opportunities for enhancements aimed at improving both safety and profit potential. The text explicitly states that because of DCS, processes can be controlled remotely, leading to a better understanding of how they operate and how to improve them, ultimately contributing to both increased safety and potential profit. The capacity to model these processes provides a critical tool for analysis, optimization, and prediction, leading to safer and more efficient operations. By providing comprehensive data and visualization tools, DCS enables more effective decision-making and enhances a company's ability to optimize its processes for maximum productivity and reduced risk.
III.Process Control Failures Case Studies
Major industrial accidents like Three Mile Island (TMI-2) and Bhopal demonstrate the severe consequences of process control failures. While TMI-2, though narrowly avoiding a catastrophe, emphasized the importance of robust control systems, the Texas City refinery explosion in 2005, which killed 15, highlighted the need for improved safety systems, operator training, and process design. These incidents underscore the ethical responsibility of engineers to ensure process safety and proper operation.
1. Three Mile Island TMI 2 Accident
The section uses the Three Mile Island (TMI-2) nuclear power plant accident as a case study to illustrate the consequences of process control design failures. The accident, while largely contained, is analyzed to highlight how a control design failure prevented operators from effectively cooling the reactor core. This failure resulted in the melting of fuel rods and the nuclear fuel itself. The severity of this near-catastrophe underscores the paramount importance of robust and reliable control systems in preventing potentially catastrophic incidents within nuclear power plants. The text highlights the averted disaster and notes a comparison with the Chernobyl disaster, where the outcome was far more devastating. This near miss underscores the necessity for stringent design and safety standards in the nuclear power industry, demonstrating the crucial role of effective process control systems in preventing major accidents.
2. Texas City Refinery Explosion
The Texas City refinery explosion in March 2005 is presented as another critical case study, illustrating the devastating consequences of process control failures. The explosion, at the third-largest refinery in the United States, resulted in 15 fatalities. A detailed account of the events leading up to the explosion reveals a series of oversights, including the failure to open a discharge valve, the disregard of high-level alarms, and inadequacies in the safety relief system. The analysis directly points to failures in written start-up procedures, insufficient operator training, and flaws in the design of the safety relief system as the root causes of the tragedy. The significant loss of life emphasizes the profound impact that shortcomings in process control can have, underscoring the critical need for comprehensive safety protocols and robust system designs. The capacity of the refinery to process over 400,000 barrels of crude oil daily is noted, highlighting the potential magnitude of catastrophic failures in large-scale industrial operations. The case underscores the paramount importance of investing in comprehensive safety measures, thorough training for personnel, and rigorous system design review as critical safeguards against similar incidents.
3. The Broader Ethical Implications of Process Control
This subsection emphasizes the broader ethical implications for engineers involved in large-scale process control. The analysis highlights that the responsibilities of engineers in this field extend far beyond the scope of their employing company, impacting surrounding communities and the environment. The catastrophic consequences of process control failures in incidents like those at Three Mile Island and Texas City underscore the critical nature of this responsibility. The text emphasizes the importance of safe and proper operation and advocates for proactive measures to improve the safety of process environments. It is stated that engineers have an important ethical responsibility to operate a process safely and properly, extending beyond their company's interests. The analysis underscores the need for a heightened sense of accountability, emphasizing that a commitment to safety is not merely a matter of compliance but a fundamental ethical obligation that directly affects human lives and environmental well-being.
IV.Process Control in Everyday Life
The principles of process control are evident in everyday activities. Examples include adjusting soup spice levels (level control), regulating water temperature in a bath (temperature control), and managing food intake to satisfy hunger (predictive control). These everyday parallels illustrate the broad applicability of process control concepts.
1. Spicing Up Soup An Analogy for Composition Control
This subsection uses the everyday task of seasoning soup to illustrate the concept of composition control in process engineering. Pavlo LaBalle, after a long day of work, uses his sense of taste (analogous to a composition sensor) to determine that his soup lacks sufficient spice. The desired level of spiciness represents the setpoint for the process. The act of adding more spice to achieve the desired level demonstrates feedback control, adjusting the input (spice) to meet the desired output (spice concentration in the soup). This simple action mirrors the fundamental principles of process control, highlighting the constant adjustment and monitoring needed to reach and maintain the setpoint in a process. The analogy effectively connects the theoretical concept of process control with a familiar everyday experience. This simple example of adjusting the spice level in soup to reach a desired setpoint helps to demonstrate the core principles of composition control in an easily understandable way, connecting theoretical concepts to real-world applications.
2. Grocery Shopping Predictive Control
This example uses grocery shopping as an analogy for predictive control. Rachel, faced with a wide selection of food, needs to predict how much food to buy to satisfy her hunger without overbuying or underbuying. Her past experiences (memory) act as a predictive control system, influencing her purchasing decisions. Her decision to buy chips, an apple, and a bagel demonstrates the application of past data to predict future needs. This exemplifies predictive control, where previous experiences inform current actions to reach a desired outcome (feeling full without excess food). Successfully purchasing the right amount of food without needing additional purchases mirrors the functionality of a predictive control system which anticipates process changes and adjusts the input accordingly. This common experience of shopping for groceries is used effectively to demonstrate the concept of predictive control, a more sophisticated control approach. The scenario helps the reader relate to the complex concept of predicting future system behavior using past data, a key aspect in advanced process control strategies.
3. Filling a Bathtub Flow Rate Control
The example of filling a bathtub illustrates the concept of flow rate control. Lan Ri, tired from working on a project, decides to take a bath. He encounters two flow rate controllers: one for hot water and one for cold water. The act of adjusting these controllers to achieve the desired water temperature and fill level demonstrates the use of flow rate controllers in achieving a desired outcome. This everyday task provides a relatable illustration of the use of flow rate controllers. The adjustment of the hot and cold water flow rates to achieve a comfortable bath temperature mimics the manipulation of flow controllers in industrial processes, where adjustments are made to meet specified targets. The story demonstrates how we implicitly use flow rate control principles to achieve a desired result, making a complex industrial concept more accessible and easily understandable for a wider audience.
V.Process Control Design and Optimization
Effective process control design involves careful consideration of customer needs, operational constraints (equipment limitations), safety constraints (company policies and regulations), environmental regulations (e.g., EPA guidelines), and economic factors. Achieving all objectives, while optimizing for cost and efficiency, is paramount. Energy management, particularly in processes with exothermic or endothermic reactions, is a critical factor affecting both cost and safety.
VI.Control Limits Setpoints and Data Analysis
Precisely defining 'equal' and 'zero' for control limits is crucial. Choosing appropriate setpoints and ranges for controllers is essential for effective process control. Data analysis tools such as ANOVA in Excel and other statistical process control (SPC) methods are used to analyze data, identify trends, and optimize processes. Properly characterizing noise (e.g., white noise, crackling noise) is critical for robust modeling and control.
1. Defining Control Limits and Setpoints
This section addresses the critical task of defining control limits and setpoints. It emphasizes that due to the high precision of modern electronics, true equality ('equal') and zero ('zero') are not achievable, necessitating the definition of acceptable tolerances. The selection of setpoints for controllers requires careful consideration, along with the acceptable range of fluctuation before corrective actions are initiated. The text highlights the importance of clearly defining these parameters to ensure the effective operation of control systems and illustrates the concept with a practical example involving a heat exchanger, where operational and safety parameters must be defined to prevent equipment damage or injury. These defined limits and setpoints act as boundaries within which the system is allowed to operate before corrective actions are required, thus ensuring safe and efficient functioning of the control system.
2. Data Analysis using Excel ANOVA and Other Techniques
This subsection describes the role of data analysis in process control, focusing on the use of Microsoft Excel. The use of ANOVA (Analysis of Variance) for comparing continuous measurements to determine if they originate from the same or different distributions is explained. This statistical technique is particularly useful when dealing with multiple samples or groups of data, helping to distinguish significant variations and potential sources of process inconsistencies. Excel's capabilities in handling various data analysis tasks, such as calculating the frequency of value occurrences and determining the sum of squared differences (residuals), are briefly mentioned. The use of the Excel Solver function is mentioned for optimizing model parameters by minimizing the sum of squared differences. This highlights the importance of employing statistical tools to analyze collected data, identify anomalies, and ultimately improve the accuracy and reliability of process control models. The ability to efficiently conduct data analysis through Excel allows engineers to make data-driven decisions which optimize process control.
3. Characterizing Noise in Process Data
This section highlights the significance of characterizing noise in process data for improving process understanding and developing optimal control strategies. The text outlines two major categories of noise: frequency-based noise (classified by 'colors' of noise, such as white noise), and non-frequency-based noise (including pops, snaps, and crackles). The concept of spectral density is introduced as a means to classify frequency-based noise, showing how signal power varies with frequency. The distinction between white noise and Gaussian white noise is explained, emphasizing that white noise indicates equal power distribution over time, while Gaussian white noise additionally implies a Gaussian probability density function for the signal. The challenges of characterizing non-frequency-based noises like pops, snaps, and crackles are briefly discussed, highlighting the relatively nascent state of research in this area. Understanding the characteristics of noise in the system is essential for designing effective control strategies and for accurately interpreting data.
VII.Modeling and Simulation using Excel and Mathematica
Excel and Mathematica offer powerful tools for process modeling and simulation. Excel facilitates basic modeling and data analysis using functions like FLOOR, FREQUENCY, and the Solver tool for optimization. Mathematica, with its DSolve and NDSolve functions, allows for more complex ODE modeling and simulations, including the handling of dead time in processes. Both tools are critical for design, testing, and optimization of control systems.
1. Excel for Process Modeling and Simulation
This section details the use of Microsoft Excel for process modeling and simulation. While acknowledging that Excel might be less accurate than dedicated modeling software, it offers a valuable tool for gaining an understanding of process behavior. The text describes its use with examples of specific functions: FLOOR (rounding numbers down), and FREQUENCY (calculating value occurrences within ranges). The process of preparing data for use with the Solver function is outlined, including organizing independent and dependent variables, calculating predicted values using model equations, and computing the sum of squared differences (residuals) to assess model fit. The use of ANOVA (Analysis of Variance) in Excel for data analysis purposes is also mentioned, highlighting its role in comparing continuous measurements to determine if they come from the same or different distributions. The simplicity and accessibility of Excel makes it a valuable tool for engineers seeking a basic yet effective approach to process modeling and simulation.
2. Monte Carlo Simulation in Excel
Within the Excel modeling section, the use of a random number generator for Monte Carlo simulations is discussed. The example of generating a random number to determine an outcome (A or B, with specified probabilities) illustrates a stochastic method. The key characteristic of such a method is the independence of each step from the previous ones. The application of the IF function and the RAND function within Excel are highlighted to perform these types of simulations. The ability to use the sort function in Excel for probability calculations is also mentioned, providing a method to find the number of data points above a specific threshold and thus estimate the probability of exceeding that threshold. This discussion demonstrates the potential of Excel in performing more advanced simulations such as stochastic modeling methods, broadening its utility beyond basic data manipulation and analysis. The simplicity of implementation in Excel makes it accessible even to users with limited programming experience.
3. Mathematica for Process Modeling and Simulation
This section introduces Mathematica as a more powerful tool for process modeling and simulation, especially for handling more complex scenarios. It mentions that Mathematica recognizes and handles common mathematical constants such as Pi, E (Euler's constant), and I (imaginary unit). The use of DSolve and NDSolve functions for solving ODEs is highlighted, with DSolve used to find general solutions and NDSolve employed when initial conditions are specified. The importance of using two equal signs ('==') to denote equality within equations in Mathematica syntax is pointed out. The text further explains the process of defining and testing user-defined functions within Mathematica, which are particularly useful for repetitive calculations where only variable values change. The section also mentions the value of the Mathematica Documentation Center as a helpful resource for navigating the program's syntax and functions, making it a comprehensive resource for engineers working with ODEs and more complex mathematical models.
VIII.Numerical Methods for Solving ODEs and Systems of ODEs
Numerical methods, such as Euler's method and higher-order Runge-Kutta methods, are crucial for approximating solutions to ordinary differential equations (ODEs) used in process modeling. Choosing the right method and step size is crucial for balancing accuracy and computational efficiency. These techniques extend to solving systems of ODEs which represent more complex real-world scenarios in chemical engineering. Managing discretization errors is important for accurate results.
1. Numerical Methods for Solving ODEs
This section discusses numerical methods for solving ordinary differential equations (ODEs), a common task in process modeling. It emphasizes that in many real-world applications, simply having a method for solving a single ODE is insufficient; often, systems of ODEs must be solved simultaneously. The text notes that the numerical methods described (Euler's method and higher-order Runge-Kutta methods) remain applicable for systems of ODEs, though the process becomes more complex, often requiring the use of software like Excel for practical application. The choice of method depends on the situation and the desired accuracy. The Euler method offers a simpler, faster solution for quick estimates, while higher-order methods like the fifth-order Runge-Kutta method provide increased accuracy but at a higher computational cost. The concept of discretization error (truncation error) and its propagation through successive steps in numerical approximation is explained. This error, inherent in numerical methods, can be reduced by decreasing the step size, but doing so increases the computational burden. The document highlights the trade-off between accuracy and computational efficiency in selecting an appropriate numerical method and step size.
2. Euler s Method for Systems of ODEs
This subsection focuses on extending the application of numerical methods to systems of ODEs, a common scenario in chemical engineering. It explains that while the fundamental principles of methods like Euler's method remain the same, solving systems of ODEs necessitates using multiple initial values for each ODE. The text states that this approach significantly increases complexity, making the use of software like Excel almost essential for practical calculations. The use of uniform time steps is discussed, acknowledging that this approach is suitable for some cases but not universally applicable. The section mentions that varying time steps may be more appropriate for certain problems, and that the choice of step size can be guided by comparing the results of different order numerical schemes, using the difference between the results as an estimate of the error. This approach allows engineers to adjust the step size to meet desired accuracy requirements, though it increases computational effort.
3. Dead Time and its Impact on Modeling
This subsection introduces the concept of 'dead time' (or delay) in process modeling. Dead time refers to the delay or lag in a real-life process, meaning that the numerical model's predictions are inaccurate until the delay has elapsed. The text explains this with the example of a CSTR (Continuous Stirred Tank Reactor), where it takes time for reagents to be discharged after the reaction starts. The dead time is defined as the period before model predictions align with the theoretical equation. Dead time can be determined experimentally and incorporated into model equations. The impact of dead time on modeling is shown to be a horizontal shift in the model equation. In Excel, this is handled by substituting 'x' with '(x-t)', where 't' represents dead time. The accurate representation of dead time in a model is essential for generating realistic simulations and predictions, particularly when dealing with dynamic systems.