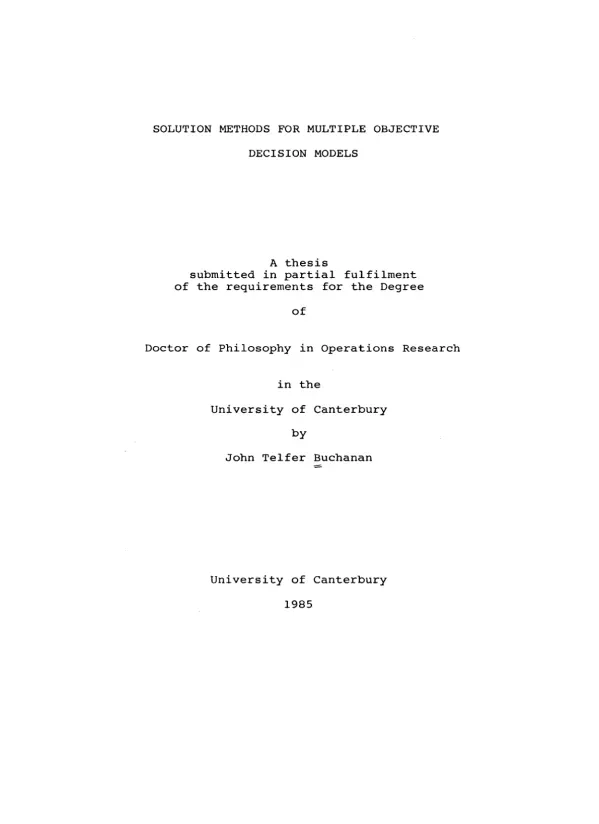
Multiple Objective Decision Models
Document information
Language | English |
Format | |
Size | 9.62 MB |
Summary
I.A Historical Overview of Multiple Objective Decision Making MODM
This section traces the evolution of MODM from its origins in the early 1950s. Early management science largely avoided the complexities of multiple objectives, preferring single-objective models often based on dollar value. A significant contribution was goal programming (GP), introduced by Charnes and Cooper (1961), which reduces multiple objectives to a single one by minimizing deviations from target levels. The rise of multi-attribute utility theory further shaped the field, leading to the development of more sophisticated MODM techniques.
1. Early Developments and the Rise of Goal Programming
Starr and Zeleny (1977) provide a foundational overview of Multiple Objective Decision Making (MODM) within management science, highlighting its emergence in the early 1950s. Initial contributions laid the groundwork for a significant advancement: goal programming, introduced by Charnes and Cooper (1961). This technique addresses the challenge of multiple objectives by transforming them into a single objective function. It achieves this by minimizing deviations between each objective and its pre-defined target level or goal. This innovative approach proved highly influential in the subsequent development of MODM methodologies. The decade following saw the extension of traditional utility theory into multiattribute utility theory, further enriching the theoretical basis for MODM. Johnsen's 1968 study, focusing on the multigoal nature of firms, is cited by Starr and Zeleny as a crucial step in recognizing the inherent complexity of real-world decision-making situations which often involve more than one objective. The integration of these diverse strands of thought provided a solid basis for further developments in the field.
2. The Prevalence of Single Objective Models and the Delayed Growth of MODM
In the early days of management science, particularly concerning practical applications, decision problems with multiple objectives were largely avoided. The preference was for simpler, single-objective models, primarily using dollar value as the unit of measurement. This approach, often implemented within linear programming models, yielded numerous successful applications, stimulating further research in this area. However, this early focus on single-objective models likely hindered the development of Multiple Objective Decision Models (MODM). The emphasis on a single, easily quantifiable objective – often monetary value – limited the scope of analysis and delayed the widespread adoption of more nuanced methods capable of handling the inherent complexities of multi-objective problems. This historical context underscores the relative novelty of MODM and the challenges in transitioning from established single-objective methods to more comprehensive frameworks. The thesis focuses on this later development, examining scenarios where decision alternatives are defined explicitly through constraints, with a specific focus on linear decision models.
3. Early MODM Assumptions and the Shift Towards Realism
Early work on MODM often relied on the assumption of an 'ideal' decision-maker (DM) who consistently acted according to their utility function. This allowed for the development of elegant solution methods demonstrating convergence to solutions maximizing utility. However, recent research increasingly emphasizes the need for solution methods that better align with the actual decision-making behavior of real-world DMs, moving beyond the traditional utility approach. This shift towards greater realism acknowledges the inherent limitations and complexities of human decision-making, recognizing that decision-makers may not always act rationally or consistently according to a predefined utility function. The initial focus on idealized models, while helpful for theoretical understanding, neglected the practical realities of how individuals and organizations approach complex decision scenarios involving multiple, potentially conflicting, objectives. This paved the way for the development of more behavioral approaches, accounting for the complexities and inconsistencies often inherent in human decision-making processes.
II. MODM Solution Methodologies A Review
This section reviews various MODM solution methodologies found in the literature. It focuses on methods where decision alternatives are explicitly stated as constraints, particularly within a linear decision model. The discussion includes an overview of different approaches, highlighting the key differences and challenges in each method. These include interactive methods, goal programming (GP), and the use of utility or value functions to represent decision-maker preferences. The challenges of incorporating realistic decision-maker behavior, going beyond the idealized 'rational' decision maker, are also discussed.
1. The Nature of MODM Solutions and the Role of Utility Functions
The section establishes the fundamental nature of solutions within Multiple Objective Decision Making (MODM). It highlights that, unlike single-objective problems, the solution to an MODM is a set of efficient or non-dominated solutions. This arises because comparisons must be made across different and often incommensurable objectives; if objectives were commensurable, the problem could be reduced to a single-objective one, as in traditional cost-benefit analysis. The inherent subjectivity in identifying the most preferred solution is emphasized, with the decision-maker's (DM's) preferences significantly impacting both the solution method and the results. Classical economics posits that a rational DM is a utility maximizer; utility theory assumes a scalar measure of preference exists for each feasible solution. However, the inherent subjectivity of preference and the limitations of utility theory in fully capturing the complexities of human decision-making are underscored as limitations of relying solely on utility maximization.
2. Goal Programming and its Criticisms
Goal programming (GP), introduced by Charnes and Cooper (1961), is presented as a key methodology in MODM. GP simplifies multiple objectives into a single objective function by minimizing deviations from pre-specified target levels. The text draws a parallel between GP and the early FORTRAN computer language, highlighting their pioneering status and subsequent critiques. Criticism of GP includes the burden on the DM to provide realistic goals, and the possibility of generating inefficient solutions (as noted by Zeleny, 1982). While GP provides a practical method for handling multiple objectives, the inherent difficulties in defining and weighting goals are emphasized. The reliance on the DM to specify precise goals a priori might be unrealistic in many complex decision situations, and the potential for suboptimal outcomes is a significant concern. The discussion sets the stage for exploring alternative approaches that address these limitations.
3. Interactive Methods Steuer s Cone Approach and the Reference Point Method
The review shifts to interactive methods for solving MODMs, emphasizing iterative processes involving the decision-maker. Steuer's method, using a 'cone of light' to narrow down the search space on the efficient surface, is explained. This interactive approach allows the DM to progressively refine their preferences based on visualizations of the solution space. The method starts by illuminating the entire efficient surface and then narrows it down as the DM provides more input. The process is repeated until a satisfactory solution is found. The limitations, such as the generation of only extreme point solutions in its initial form, are also discussed. Another interactive approach is Wierzbicki's reference point method. This method assumes the DM has certain aspiration levels which are used as reference points for iteratively finding attainable efficient solutions. The iterative nature of these methods highlights the need for effective communication and collaboration between the analyst and the DM, adapting to the DM's evolving preferences throughout the solution process.
4. A Posteriori Articulation of Preferences and Other Methods
The section addresses methods where the decision-maker provides information after the generation of solutions, noting that finding all efficient solutions is often impractical due to their potentially infinite number. Therefore, these methods often focus on finding efficient extreme-point solutions. The section also touches upon other interactive methods including the work of Monarchi et al. (1973), which uses surrogate objective functions defined by the DM's aspirations, allowing for interval goals rather than fixed points. The challenges inherent in aggregating individual preferences into a group preference relation are mentioned, highlighting Arrow's Impossibility Theorem. The section concludes by acknowledging the existence of other interactive methods while emphasizing that the major concepts have been covered, setting the stage for the subsequent discussion of the practical limitations and the need for a more behavioral approach.
III.Behavioral Issues in Decision Making and Their Implications for MODM
This section examines the empirical evidence regarding human decision-making behavior and its implications for MODM solution methods. It contrasts the normative assumptions of classical decision theory, which assumes an idealized decision-maker, with the concept of bounded rationality, acknowledging human limitations and environmental uncertainty. The section explores how actual choice behaviors often deviate from the axioms of expected utility theory and discusses how these findings necessitate a more realistic approach to MODM solution methods. The influence of factors like ambiguity and the number of dimensions considered simultaneously are also examined.
1. Defining Rationality and the Concept of Bounded Rationality
The chapter begins by addressing the complex question of what constitutes rational behavior in decision-making. It contrasts two definitions: one where rationality is judged by the means used to achieve a goal, and another where rationality is defined by the goals themselves. Zeleny's (1976) definition, aiming to be as close as possible to a perceived ideal, is presented as a means-oriented approach. This leads to the introduction of Herbert Simon's (1955, 1957) concept of bounded rationality. This concept acknowledges that decision-making occurs within constraints imposed by human limitations and the complexities of uncertain environments. Simon argues that human decision procedures, while sensible within these constraints, might appear less so if those constraints were removed. This contrasts with classical decision theory, which assumes an idealized decision-maker whose preferences perfectly align with the axioms of utility theory. The implications are that methods appearing sensible in theory might prove less so in practice, setting the stage for examining empirical evidence of human choice behavior.
2. Empirical Evidence Contradicting Expected Utility Theory
This section presents empirical evidence demonstrating that human choice behavior frequently contradicts the predictions of expected utility theory. The discussion highlights the persistent gap between theoretical prescriptions and observed decision-making processes. While numerous studies show discrepancies, the lack of strong predictive power is noted, using Wallsten's (1980) observation that these studies often 'analyze the scratch but not the itch'. Modeling efforts have largely focused on describing the judgment policies of decision-makers rather than predicting precise choices. An example illustrating the limitations of simplified models in representing complex decision-making processes is given. The persistent inconsistencies between human behavior and the predictions of expected utility theory necessitate a more nuanced understanding of decision-making processes. This includes exploring various decision strategies (compensatory and non-compensatory) and how these can be incorporated into more realistic models.
3. Judgmental Inconsistency and the Role of the Environment
A key challenge in modeling human decision-making is the observed inconsistency in applying decision strategies. Oudycha and Naylor (1966) highlight this, suggesting that humans often generate correct strategies but fail to use them consistently. Their proposed solution involves capturing the judgment policy of the DM and then implementing it through a machine. The root of this inconsistency remains unclear but is likely linked to the human limitations discussed earlier and the uncertainty inherent in the environment. The role of the environment in shaping judgment is discussed, referencing Brunswik's Lens Model and Bell's (1982) argument that management science needs to accommodate environmental pressures in its analysis. Further, the limited capacity of humans to process information simultaneously (Miller's 1956 suggestion of about seven dimensions) is discussed, indicating the need for decision-making models that account for such limitations and for different types of strategies that may be used sequentially to simplify the decision-making process.
4. Social Judgment Theory and Implications for MODM Solution Methods
Social Judgment Theory (SJT) is presented as an approach to modeling choice behavior, particularly in group decision-making situations. The discussion touches upon the challenges of aggregating individual preferences, citing Arrow's (1951) Impossibility Theorem and the voting paradox. While expected utility theory remains valuable due to a lack of better alternatives, the chapter emphasizes that MODM solution methods should not penalize DMs for behavior inconsistent with utility theory axioms. The limitations of relying solely on utility-based approaches and the advantages of methods that incorporate environmental factors are highlighted. The chapter concludes that although human behavior may appear irrational at times, it is intelligent within the bounds of its limitations and environmental uncertainty, leading to bounded rationality. Rather than correction, human decision-making requires support and aid, guiding future research towards better aligning methods with the characteristics of real-world decision-makers.
IV.An Experimental Comparison of MODM Solution Methods
This section details an experiment comparing four different MODM solution methods: Zionts-Wallenius (ZW), Steuer (STE), a naive method, and SWT. The experiment used a case study of a fictitious New Zealand manufacturing company producing electrical components for lamps, modeled using a multi-objective linear programming (MOLP) problem with three objectives: minimizing operating costs, stockouts, and temporary layoffs. The results showed statistically significant differences in user preference and confidence levels across the various methods, particularly highlighting the trade-offs between efficiency, ease of use, and decision-maker control. The ZW method, despite its efficiency, received lower confidence scores than others. The naive method, while simple, yielded surprisingly good results and showed that a balance of control and guidance is needed.
1. Experimental Design and Case Study
This section describes an experiment designed to compare four different Multiple Objective Decision Making (MODM) solution methods: Zionts-Wallenius (ZW), Steuer (STE), a naive method, and a method referred to as SWT. The experiment utilized a case study focusing on a small, fictitious New Zealand manufacturing company producing electrical components for lamps. This case study, adapted from Wallenius's work, was presented to subjects in advance. A linear programming model with three objectives was developed to represent the company's production scheduling problem: minimizing operating costs, the average number of stockouts, and the average percentage of the labor force temporarily laid off. Before the experiment began, subjects were questioned to ensure they understood the case study. A distinction between efficient and inefficient solutions within the context of the multi-objective linear programming (MOLP) model was explained. A graphical representation of each solution method was also presented to subjects prior to use, providing clear instructions for participation.
2. Methodological Differences and Expected Convergence
The section highlights key differences between the four MODM solution methods. The Zionts-Wallenius (ZW) method relies on pairwise assessments of trade-offs, while the Steuer (STE) method requires the DM to choose among six solutions at each iteration. In terms of convergence to a most preferred solution, both ZW and SWT are expected to converge under an ideal DM, as is STE. The naive method, however, is not designed to converge in any predetermined fashion. Regarding termination, ZW and SWT terminate internally while STE and the naive method terminate externally. STE is characterized as a global approach, starting from outside the solution space to find a preferred solution. In contrast, ZW and the naive method represent local approaches, meaning the OM doesn't get to see the complete picture; SWT falls somewhere in between these two approaches. These contrasting features are crucial in understanding the diverse strategies employed and their potential impact on user experience and the effectiveness of achieving an optimal decision.
3. Experimental Results and Statistical Analysis
The results of the experiment, conducted in a controlled setting, are presented and analyzed. The Steuer method (STE) was found to be significantly preferred over the other three methods at a 5% significance level. Subjects expressed significantly less confidence in the Zionts-Wallenius (ZW) method than in the other methods, a finding also significant at the 5% level. This lack of confidence is attributed to the relatively short time subjects spent on the ZW method (median 5.3 minutes), suggesting a lack of familiarity. The significance of differences across methods regarding elapsed time is discussed, although the impact on practical decision-making scenarios where a solution might take 20 minutes is deemed to be marginal. However, if frequent solutions were required, the differences might become significant, making the ZW method potentially more desirable due to its speed. Further statistical tests (Mann-Whitney U and Kruskal-Wallis one-way ANOVA) were performed, showing that apart from SWT (where earlier assessments were more favorable), there were no significant effects due to position in the experiment.
4. Discussion of Methodological Preferences and a Hybrid Approach
The section delves into the reasons behind the observed preferences for different methods. The relative lack of confidence in the ZW method is further explored, suggesting that its efficiency may come at the cost of decision-maker autonomy. The comment 'I feel as if I have just purchased something from a fast-talking salesman' captures this sentiment. Subjects who value substantial control or those who prefer more guidance from a method exhibited different preferences. The surprisingly good performance of the naive method is analyzed, linking preference to the decision-makers' desire for control versus the need for direction. A hybrid approach, combining the Steuer method and the naive method, is suggested based on subject feedback. This combined method aims to leverage the strengths of both—the initial exploration of solutions with the naive method followed by the refined search with the contracting cone approach of the Steuer method, showing that sometimes a combination of methods would be more effective for a user.
V.New Solution Methods and Tradeoff Information in MODM
This section introduces two new solution methods and examines the critical issue of providing effective tradeoff information to decision-makers. The use of dual variables to derive tradeoff information is explored, along with methods to manage situations where linear dependencies (LDF) exist amongst objectives. The concept of a symmetrical linkage system, an analytic approach for linking social values with scientific information using SJT, is discussed, showing how MODM is applied in broader real-world contexts such as regional air pollution problems (e.g., the Denver air pollution case study using simulation models). The development of the Tradeoff (TO) method, which provides local tradeoff information, is highlighted as a means to improve the iterative refinement process in methods where decision making is less than completely rational.
1. The Need for Effective Tradeoff Information
This section emphasizes the critical role of providing decision-makers with clear and useful tradeoff information within Multiple Objective Decision Making (MODM) methods. It notes that while pairwise tradeoff information is readily available in some methods, total tradeoff information is less accessible. The challenge is highlighted in situations involving linear dependencies (LDFs) among objectives. At an LDF solution, ratios of dual variables from binding objective constraints provide valid tradeoff information for a unit change in one objective relative to another. However, tradeoffs affect all objectives, and the magnitude and direction of changes in non-binding constraints cannot be directly ascertained using standard formulations. The need for more sophisticated methods to provide comprehensive tradeoff information, especially in scenarios with multiple objectives, is underscored as crucial for informed decision-making. The difficulty in providing this information, especially for larger problems, is a key limitation for many methods. This sets the stage for the introduction of new methods that aim to address this deficiency.
2. Introduction of the Tradeoff TO Method and its Similarities to the Reference Point Approach
The section introduces the Tradeoff (TO) method, designed to provide local tradeoff information at each achieved solution, making the iterative refinement process easier and facilitating better decision-making. The TO method is compared to the reference point approach (Lewandowski and Grauer, 1982). Both methods utilize a scalarizing function where weights are determined by the decision-maker's (DM's) specified reference point or desired solution. Each provides a vector of dual variables at the optimal solution from which tradeoff information can be derived. However, the TO method emphasizes pairwise tradeoffs, guiding the DM across the efficient surface. The reference point approach, in contrast, doesn't explicitly detail how the dual vector is used beyond guiding the DM's selection of subsequent reference points. The similarities highlight the potential for improved decision support through methods which provide more explicit tradeoff information and iterative interaction with the decision maker.
3. Addressing Limitations of the Tradeoff Method and the Role of Social Judgment Theory
The section acknowledges limitations of the TO method in its current form. Specifically, without extensions, it is not ideally suited for MODMs with a large number of linear dependencies (LDFs). However, the author notes that LDF situations are relatively uncommon in MOLP models used for research, and the TO method is particularly well-suited to models with only two objectives. The applicability in real-world settings, especially in the New Zealand context cited by Read (1985), is also considered. A broader perspective on linking social values with scientific information is introduced through the discussion of Social Judgment Theory (SJT). Mumpower et al. (1979) highlight the 'Symmetrical Linkage System' of SJT, which gives equal weight to social values and scientific information. The application of SJT to the Denver regional air pollution problem demonstrates its usefulness in scenarios where multiple stakeholders and values are involved, further expanding the scope beyond traditional technical optimizations within the MODM context.