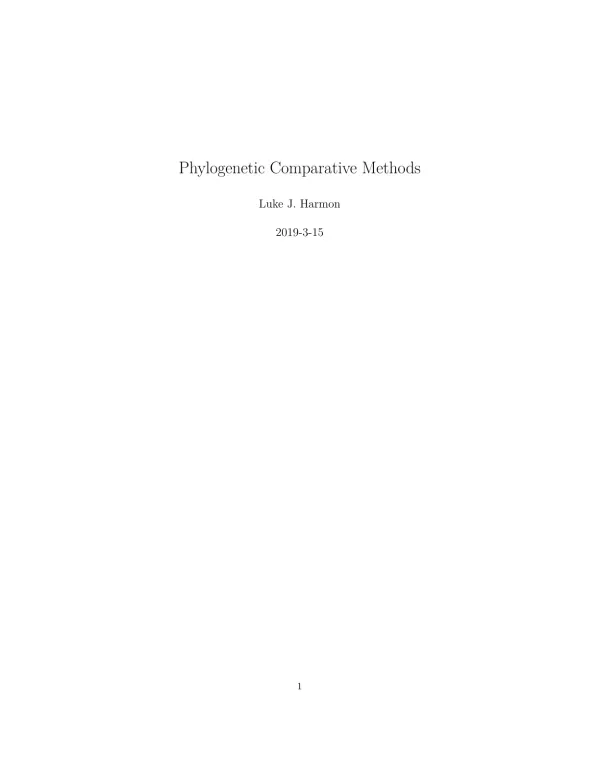
Macroevolutionary Research: Models & Data
Document information
instructor/editor | Arne Mooers |
subject/major | Evolutionary Biology |
Document type | Book |
Language | English |
Format | |
Size | 12.24 MB |
Summary
I.Phylogenetic Comparative Methods Connecting Evolutionary Processes to Broad Scale Patterns
This book explores phylogenetic comparative methods, using phylogenetic trees to understand large-scale evolutionary patterns. It focuses on connecting evolutionary processes (like genetic drift, selection, and speciation) with the observed diversity of life. The core methodology combines biology, mathematics, and computer science, leveraging tree of life data to infer past events and understand the forces shaping biodiversity. Key challenges include accounting for uncertainty in phylogenetic trees (gene trees vs. species trees) and developing methods to handle increasingly large datasets.
1. The Tree of Life and Phylogenetic Comparative Methods
The section introduces the concept of the tree of life as a fundamental tool in understanding evolution. It highlights the tree's role in illustrating relationships between species, identifying exceptionally diverse or depauperate groups, and tracing the evolution and spread of life across the globe. The book focuses on phylogenetic comparative methods, which use phylogenetic trees and associated data to investigate evolutionary processes. These methods combine biology, mathematics, and computer science, allowing researchers to study a wide range of evolutionary questions. Examples of such questions include determining the prevalence of various evolutionary processes across different clades, comparing evolutionary patterns across lineages, and evaluating if current evolutionary mechanisms are sufficient to explain observed biodiversity or if additional, rarer processes are needed (e.g., adaptive radiation, species selection). The limitations of relying solely on the current species are also acknowledged, emphasizing the importance of incorporating methods which account for uncertainty inherent in phylogenetic tree estimation. References to Rosindell and Harmon (2012) and Baum and Smith (2012) provide further reading on the tree of life and its applications in evolutionary biology. The limitations of current techniques in handling increasingly large phylogenetic trees are also discussed.
2. The Role of Population and Quantitative Genetics
This section emphasizes the foundational role of population and quantitative genetics in understanding evolutionary processes. Models from these fields are described as central to evolutionary biology and closely related to many comparative methods. While population genetics primarily focuses on allele frequencies and quantitative genetics on traits and heritability, genomics is blurring this line. The text traces the roots of these approaches to the modern synthesis, citing the work of Fisher (1930) and Wright (1984), while also mentioning later elaborations (Falconer et al., 1996; Lynch and Walsh, 1998; Rice, 2004). Although often applied to short-term evolutionary changes, these models are also valuable in macroevolutionary studies. Lande's (1976) work on quantitative genetic predictions for trait evolution using Brownian motion and Ornstein-Uhlenbeck models is highlighted as a significant contribution. The section also points out that existing models sometimes predict rates of evolution that are too fast, indicating the need for more refined approaches. Further study and discussion regarding Pennell and Harmon (2013) is suggested for a more comprehensive understanding of the intersection of these fields with comparative methods.
3. Uncertainty in Phylogenetic Trees and Methods for Addressing It
A crucial point is made regarding the inherent uncertainty in phylogenetic tree estimations. It is argued that assuming perfect knowledge of a phylogenetic tree is unrealistic, given the difficulties in estimating branch lengths accurately from limited genetic data. The differences between gene trees and species trees are also highlighted as a source of error. The text advocates for comparative methods that explicitly account for this uncertainty in both tree topology and branch lengths. While the chapter does not offer concrete solutions at this stage, the section lays the groundwork for the later chapters' introduction of methods which aim to address this crucial issue. The rapidly increasing size of phylogenetic trees, with tens of thousands or even millions of tips in the near future, poses a significant challenge for current visualization and analysis techniques. The need for new methodologies to extract meaningful information from these massive datasets is emphasized, highlighting a significant practical concern for the field.
4. Limitations of Phylogenetic Trees and the Importance of Model Selection
This section discusses the limitations of phylogenetic trees, emphasizing that they don't provide all the answers in evolutionary studies. Specific limitations mentioned include the challenges in reconstructing ancestral traits (ancestral state reconstruction, Losos 2011), distinguishing between models with varying evolutionary tempos (Slater et al., 2012a), and reliably detecting extinction from the tree's shape alone (contrasting a viewpoint expressed by Rabosky, 2010). The text argues that while phylogenetic trees are rich sources of information, their limitations should be recognized (Alroy, 1999). The importance of constructing and testing mathematical models of evolution is stressed. The authors advocate for comparative approaches that prioritize parameter estimation and model selection over simple hypothesis testing using p-values. Such methods, they argue, should allow fitting of biologically meaningful models, while quantifying the uncertainties in parameter estimates. Model selection, the process of objectively choosing the best model from a set of possibilities using empirical data, is presented as a valuable tool for connecting statistical analyses to specific biological questions.
II. Bayesian Methods
The book contrasts frequentist and Bayesian statistical approaches. Frequentist methods rely on hypothesis testing and p-values, often focusing on Type I error control at the expense of Type II errors. Bayesian methods, conversely, provide probability estimates of hypotheses given the data, requiring the incorporation of prior knowledge. The choice between these approaches depends on the specific research question and the willingness to incorporate prior information about the data-generating model. Both approaches are useful tools in this field.
1. Frequentist Hypothesis Testing Limitations and Challenges
This section delves into the frequentist approach to statistical hypothesis testing, highlighting its emphasis on rejecting null hypotheses. The approach involves defining a null hypothesis representing the absence of a pattern or process of interest, and then assessing whether the data provide sufficient evidence to reject this null in favor of an alternative hypothesis. Examples provided include comparing the mean body size of two lizard species and investigating the relationship between body size and leg length. The concept of statistical error, including Type I (false positive) and Type II (false negative) errors, is introduced. The frequentist approach prioritizes control of Type I errors, often setting a significance level (α) of 0.05. However, the text points out the substantial limitations of this focus. The use of Bonferroni corrections for multiple testing, though intended to control Type I errors across multiple tests, drastically inflates Type II error rates. This is considered a major drawback since, as Perneger (1998) states, "…type II errors are no less false than type I errors." The authors also note that in biological contexts, null hypotheses often represent overly simplistic and unrealistic scenarios. Therefore, relying solely on hypothesis testing may not fully capture the nuances of evolutionary data. They suggest that estimating parameter values and utilizing model selection offers more informative approaches.
2. Bayesian Approaches Incorporating Prior Knowledge and Quantifying Uncertainty
The section introduces Bayesian approaches as an alternative to frequentist methods. A key advantage of Bayesian methods is their ability to directly estimate the probability of a hypothesis being true given the observed data, Pr(H|D). This is presented as more intuitive than the frequentist approach. However, Bayesian methods require the explicit quantification of prior knowledge, Pr(H), which represents beliefs about the hypothesis before considering the data. The use of "uninformative" priors, aiming to minimize their influence on the analysis, is discussed, but the complexities surrounding this concept are acknowledged. The text further emphasizes that the Pr(D) term (probability of the observed data, integrated over prior parameter distributions) is a critical element of Bayes' theorem. A central point of comparison between Bayesian and likelihood-based approaches revolves around the use of priors. The authors argue that the Bayesian assumption of having identified the true model that generated the data is often unjustified in comparative biology. This difference is linked to the contrasting philosophies of AIC and Bayesian methods; AIC assumes models are approximations of a complex reality, whereas Bayesian approaches often assume one model is correct. Consequently, AIC might favor more complex models than Bayesian methods. The superior handling of uncertainty in Bayesian analysis is highlighted as a particular strength in the context of phylogenetic comparative methods.
III.Modeling Trait Evolution Brownian Motion and Beyond
Simple models of trait evolution, such as Brownian motion, are initially presented, emphasizing their statistical advantages despite potential biological limitations. These models assume that traits evolve neutrally through genetic drift. However, the text later expands to include more realistic models of evolution which incorporate the effects of natural selection. This chapter explores different models of trait evolution under Brownian motion (pure drift, randomly varying selection, varying stabilizing selection), demonstrating that multiple models can produce indistinguishable patterns in extant species. More complex models such as the Ornstein-Uhlenbeck (OU) model are introduced to account for stabilizing selection. The estimation of the rate of evolution (often expressed as Darwins or Haldanes) is also discussed.
1. Brownian Motion Models A Simple Starting Point
The chapter introduces Brownian motion as a basic model for trait evolution. This model assumes that evolutionary change is neutral, driven solely by genetic drift, with traits changing randomly over time. A simple model is presented, assuming a character is influenced by many genes of small effect, and that the character's value does not affect fitness. While acknowledging that these assumptions might seem unrealistic for many traits (e.g., lizard body size), the authors highlight the statistical benefits of using Brownian motion models for comparative analyses. Many results are simpler under Brownian motion than with other models. Furthermore, some methods are robust to minor deviations from the assumptions of Brownian motion. Lande (1976) is cited for his early work in using Brownian motion and Ornstein-Uhlenbeck models to predict trait evolution over many generations. Lynch's (1990) finding that these models often predict long-term rates of evolution that are too fast is also discussed, highlighting the limitations of simple models when applied to long timescales.
2. Multiple Models and Brownian Motion Indistinguishable Patterns
This section explores the limitations of interpreting Brownian motion patterns. It reveals that several different evolutionary processes (pure drift, randomly varying selection, varying stabilizing selection) can produce patterns that are statistically indistinguishable from Brownian motion. Even constant directional selection can create patterns indistinguishable from Brownian motion among extant species. The authors conclude that one cannot distinguish among these models based solely on qualitative patterns of evolution. The differing Brownian motion rate parameters in these models are related to factors like population size and selection strength; knowledge of these parameters is critical for distinguishing between different scenarios. The introduction of the phylogenetic variance-covariance matrix (C) is critical, as this matrix describes the expected statistical distribution of traits on a phylogenetic tree under a Brownian motion model. This matrix is crucial for understanding the covariances of trait values based on shared phylogenetic branch lengths.
3. Fitting Brownian Motion Models and Estimating Evolutionary Rates
This section focuses on practical application, demonstrating how to fit Brownian motion models to single characters. Using mammalian body size as an example (Garland, 1992), the text explains that estimating the rate of evolution under Brownian motion requires estimating two parameters: the ancestral state at the root of the tree and the diffusion rate (σ2), which is considered the rate of evolution in comparative approaches. The importance of log-transforming data involving measurements from living organisms is also emphasized. The concept of independent contrasts, where sister taxa are compared to understand character evolution, is explained, along with a method that addresses issues arising from deeper nodes in the tree using a pruning algorithm. The text highlights the distinction between evolutionary rates estimated using Brownian motion and rates calculated from fossil data or contemporary time-series data. The latter, measured as Darwins or Haldanes, better capture evolutionary trends (Harmon, 2014), and care should be taken when comparing these different types of rates (Gingerich, 1983).
4. Beyond Brownian Motion More Complex Models of Trait Evolution
The section acknowledges the limitations of Brownian motion models and explores more complex models. The studies of Darwin's finches (Grant & Grant, 2002, 2011) are used to illustrate how natural selection, influenced by factors like rainfall and seed availability, drives trait evolution. Pagel's κ model, which allows for varying rates of character change concentrated at speciation events, is introduced but its limitations are discussed. The chapter then moves to discuss the Ornstein-Uhlenbeck (OU) model, suitable for traits evolving under stabilizing selection towards an optimal value. While this model is mathematically tractable, the authors caution against directly inferring constant stabilizing selection from the OU process alone, as other models might also fit this pattern. The inherent limitations of this simplified model, particularly over long timescales, are highlighted. In addition to specific models, general methods of modeling rate shifts and incorporating uncertainty into rate estimations are explained.
IV.Analyzing Discrete Character Evolution The Mk Model and its Extensions
This section shifts focus to discrete character evolution, using the Mk model as a basis. The Mk model describes the evolution of discrete traits on phylogenetic trees. Challenges in modeling discrete character evolution are discussed, highlighting differences between analyzing molecular sequences and other types of character data (e.g., phenotypic traits). The book mentions extensions beyond the basic Mk model, acknowledging the need for further development of methods for fitting more complex models to phylogenetic trees.
1. Discrete Character Evolution Introduction and the Mk Model
This section introduces the concept of modeling the evolution of discrete characters on phylogenetic trees, contrasting it with the previously discussed continuous character evolution. The chapter uses the example of limblessness in squamates (lizards and snakes) to illustrate the types of questions that can be addressed, noting that snakes are a limbless clade nested within squamates, losing their limbs approximately 170 million years ago (Hedges et al., 2006). The Mk model is introduced as a fundamental model for analyzing discrete character evolution. Calculating likelihoods for this model is straightforward when ancestral and nodal states are known, but in real-world scenarios, this information is usually missing. The main challenges are the unknown ancestral state at the root of the tree and the unobserved states at internal nodes. Therefore, the likelihood calculation must consider all possible combinations of character states across internal branches, making the process significantly more complex than for continuous characters. The diversity of squamates is described, highlighting the contrast between large species like the Komodo dragon (Varanus komodoensis) and small species like leaf chameleons (Brookesia) (Vitt et al., 2003; Pianka et al., 2017), emphasizing the breadth of this clade and the significance of studying the evolution of discrete traits like limblessness within this group (Streicher and Wiens, 2017).
2. Felsenstein s Pruning Algorithm and Ancestral State Probabilities
The section details Felsenstein's pruning algorithm, a method for calculating probabilities of character states on phylogenetic trees. This algorithm works backward in time from the tips to the root of the tree. At the root, the probabilities for each character state must be specified. Three different methods are presented: assuming equal probability for each state, using the model's stationary distribution, or employing prior information from fossils or outgroup taxa. The authors highlight that the first two methods are commonly used, though they can yield different results. The pruning algorithm is discussed as a valuable tool in comparative methods, highlighting its broader applicability to various phylogenetic problems. The explanation of the algorithm shows how it can be used to calculate probabilities by working back from the tips to the root. The importance of choosing an appropriate method for assigning probabilities to ancestral character states is stressed, with the choice impacting the overall results.
3. Beyond the Mk Model Challenges and Future Directions
This section addresses the limitations of simple models like the Mk model and discusses challenges in modeling discrete character evolution. The text contrasts the challenges of analyzing discrete character data with the relative ease of analyzing molecular sequence data, which typically involves thousands or millions of characters. A key difference is that sequence analysis often assumes character independence but a shared underlying model across all characters, while with discrete character data each character requires its own set of parameters. This makes fitting more complex models substantially more difficult. The example of frog reproductive strategies (Zamudio et al., 2016; Rey, 2007; Fukuyama, 1991) is used to illustrate the diversity and complexity of discrete characters in nature, ranging from the classic aquatic tadpole stage to direct development and foam-nesting strategies. The section concludes by calling for more sophisticated models, such as threshold models (Felsenstein, 2005, 2012), to better address the complexities of discrete character evolution. It highlights the need for methodological advances to keep pace with the growing amount of character data available.
V.Birth Death Models Understanding Species Diversification and Extinction
This section introduces birth-death models for analyzing species diversification and extinction. These models use the waiting times between speciation events to estimate rates of speciation (λ) and extinction (µ). The text discusses various methods for fitting these models to phylogenetic trees, including the use of lineage-through-time (LTT) plots. The impact of incomplete sampling on inferences is also noted, along with methods for correcting for this sampling bias, such as those described by Hohna and Stadler.
1. Plant Diversity and the Introduction of Birth Death Models
This section uses the dramatic diversity of angiosperms (flowering plants) – over 260,000 species – in contrast to their relatively less diverse sister groups (gymnosperms, ~1000 species; and squamates, <8000 species) to introduce birth-death models. The angiosperm clade originated over 140 million years ago (Bell et al., 2005), highlighting the rapid diversification within this group. This diversity imbalance motivates the use of birth-death models, which explicitly consider both speciation (birth) and extinction (death) rates to explain present-day diversity patterns. The chapter uses this example to emphasize that extinction rates cannot be ignored when studying diversification. An example showing diversification rates for different plant lineages (Madriñán et al., 2013) shows how estimated diversification rates depend strongly on assumed extinction rates (ϵ). Even though relative extinction rates impact absolute diversification rate estimates, their relative ordering is often robust to these assumptions. The text mentions improved methods for testing diversification rate differences across clades, referring to the richness Yule test (Paradis, 2012) as a more robust alternative to the Slowinsky and Guyer test. The example of fleshy fruits showing a significantly higher diversification rate than other clades is provided. The work of Gould et al. (1977) and Raup (1985) which spurred the development of modern quantitative macroevolutionary approaches is mentioned.
2. Estimating Birth and Death Rates Likelihood Methods and Model Selection
This section delves into the methodology of fitting birth-death models to phylogenetic trees. It explains that most modern approaches utilize the intervals between speciation events (waiting times) to estimate the model parameters, λ (speciation rate) and µ (extinction rate). Lineage-through-time (LTT) plots, which graph the number of lineages against time, are presented as a way to summarize the pattern of species accumulation in a tree. The use of maximum likelihood (ML) estimation and model selection (using AIC scores) to fit birth-death models is described. An example using the Lupinus tree (Drummond et al., 2012, with 137 species and an age of 16.6 million years) demonstrates how to estimate parameters and compare models. The example showcases how a birth-death model is preferred to a pure-birth model (based on AIC) though the evidence for extinction is not overwhelming. The importance of using all the information available in the waiting times of the tree is emphasized. The text touches upon extensions to the basic approach, mentioning Hohna’s (2011) diversified sampling model and the work of Stadler and Smrckova (2016) on likelihood calculations for representatively sampled trees and time-varying rates.
3. Beyond Constant Rates Accounting for Variation in Speciation and Extinction
This section introduces the concept of non-constant rates of speciation and extinction. The example of island archipelagos as hotspots of speciation (Losos & Schluter, 2000; Hughes & Eastwood, 2006) is used to illustrate how diversification rates can vary significantly across clades. It also points out that speciation rates are often elevated, and extinction rates reduced, following mass extinction events (Sepkoski, 1984). The contrasting diversification rates of different amphibian clades in the Pacific Northwest of the USA (Roelants et al., 2007; Jetz & Pyron, 2018) further illustrate this point. Methods for comparing the fit of models with varying speciation and extinction rates across clades are described, including a comparison of four nested models (constant rates vs. varying speciation/extinction rates in a specific clade). Model selection using AICc is highlighted as a valuable approach. The text discusses density-dependent diversification (Rabosky, 2013) and how extinction rates can change through time due to factors like climate change (Benton, 2009). The section concludes by discussing the limitations of current approaches, noting that all current models assume that rate changes occur at discrete points in the phylogenetic tree, and there is no mathematical solution for modelling changes using a Poisson process. The work of Rabosky (2017) is mentioned regarding the limitations of current models when dealing with clades that did not survive to the present day. Etienne et al.'s (2012, 2016) approach to modelling density-dependent diversification is also described.
VI.Modeling Variable Rates of Evolution Beyond Constant Rates
The final sections address the limitations of constant-rate models and explore methods to model variation in diversification rates through time and across clades. It discusses density-dependent diversification where diversification rates depend on the number of lineages present. Methods for incorporating such temporal and clade-specific rate variation into birth-death models are discussed, again acknowledging the mathematical challenges involved in creating and fitting such models. Specific examples from amphibians are provided.
1. Variable Diversification Rates Across Clades
This section acknowledges that diversification rates are not constant across all clades. The chapter begins by highlighting the uneven distribution of biodiversity across the tree of life. Island and island-like habitats are cited as examples of 'hotspots' of speciation, exhibiting exceptionally high diversification rates (Losos & Schluter, 2000; Hughes & Eastwood, 2006). The influence of mass extinctions on diversification rates is also discussed, with studies showing elevated speciation and/or reduced extinction rates following such events (Sepkoski, 1984). The authors provide a local example from the Pacific Northwest of the United States, contrasting the high diversification rates of Ranidae and Hylidae frogs with the extremely low diversification rate of Ascaphidae and Leopelmatidae frogs (Roelants et al., 2007; Jetz & Pyron, 2018). The Ascaphidae and Leopelmatidae lineages, with only six species total, are shown as the sister group to the vast majority of frog diversity (~7000 species). This showcases significant variation in diversification rates among closely related clades. Methods for formally comparing diversification rates are mentioned, including comparing models with constant rates against those where speciation or extinction rates differ for a specific clade. The use of AICc for model selection is recommended for comparing multiple, potentially non-nested, models.
2. Methods for Analyzing Variable Diversification Rates
The chapter details different approaches for modeling variable diversification rates. It explains that current methods typically model rate shifts at discrete points on the phylogenetic tree—along specific branches leading to extant taxa. The authors discuss the limitations of these approaches, noting that they are approximations that don't fully account for the possibility of rate shifts in clades that have gone extinct. The challenges in creating more sophisticated methods are discussed. The work of Moore et al. (2016) is referenced, highlighting the lack of mathematical solutions for modeling rate shifts using methods like Poisson processes, which would allow for a more precise modeling of rate shifts at arbitrary points on the tree. The section mentions the diversified sampling (DS) model by Hohna (2011) and the work of Stadler and Smrckova (2016) as alternative approaches that can handle incomplete sampling and time-varying rates. This acknowledges that our ability to infer these processes from phylogenetic data is limited by both current mathematical tools and our incomplete sampling of the tree of life.
3. Time Varying Diversification Rates Density Dependence and Other Factors
The final section addresses variation in diversification rates through time. The concept of density-dependent diversification, where diversification rates depend on the number of existing lineages, is introduced (Schluter, 2000; Rabosky, 2013). This concept is contrasted with the potential for extinction rates to vary through time, for instance due to periods of unfavorable environmental conditions (Benton, 2009). A formula for density-dependent diversification (λ(t) = λ0(1 − Nt/K)) is presented (Equation 12.8), showing how speciation rates change over time depending on the number of lineages (N) and carrying capacity (K). The methods of Etienne et al. (2012, 2016) for dealing with this type of model are described as relying on numerical solutions of differential equations. The overall method is similar to the approaches discussed by Morlon et al. (2011), but with key differences in implementation. The text also notes that an apparent slowing of diversification rates towards the present day could also be an artifact of incomplete sampling (Pybus & Harvey, 2000), emphasizing the importance of considering sampling bias when interpreting diversity patterns. Methods for addressing non-random sampling bias are mentioned (Cusimano & Renner, 2010; Brock et al., 2011), while also acknowledging that the true number of species in any clade is always uncertain and may be underestimated, especially for recently diverged lineages.